COVID was not fun
I hope this is the last thing I ever write about COVID. To help make it so, I’m leaving everything right here, posting, and not looking back or else I’ll turn into a pillar of salt. No matter how all-consuming and revolutionary a chapter of our life may be, there will always be a time to turn the page, and, for me, now is the time to put COVID to rest.
The COVID-19 pandemic was an odyssey for all 7.9 billion people who lived through it, and each person’s story shines light on this global event. As later generations will try to look at and learn from the history of COVID-19, it’s essential that we tell our stories as honestly and faithfully as we can to ensure the full complexity of this global crisis can be handed down to them.
The Big Picture: Who I am and what you’re about to read
My COVID journey followed an unexpectedly influential arc for a math guy in Bozeman. In fact, I’ve had a similarly unusual academic journey.
I was a valedictorian at the University of New Mexico with two bachelors degrees in an unusual combination of fields: biology and applied mathematics. I did many REUs in various topics, from isotope ecology (the flux of chemicals like carbon throughout food webs) and protein biochemistry (the biochemical mechanisms by which bacteria evolved antibiotic resistance), to ecoimmunology (how snail immune systems & defense against parasitic worms are disrupted by lack of food, heat, etc.) and a thesis in 2009 analyzing nonlinear dynamical systems used in epidemiology. While we’ve used math in biology since before Fibonnaci’s sequence describing the growth of rabbit populations, we’re now living through a Renaissance of quantitative biology in our age of computers, sequencing, big data, and the internet. I was a NSF-GRFP recipient, and I did my PhD in 4.5 years at Princeton’s program for Quantitative & Computational Biology, studying mathematical models of competition and discovering cool connections between models of ecological competition (trees fighting over canopy space, predators fighting over prey, microbes competing over food in our gut, etc.) and competition in economic systems (companies competing over customers, stocks in a portfolio competing over market share, etc.). My interests are weird, yet timely.
My first postdoc was set to be at Duke University, studying microbiomes or large communiteis of microbes surveyed in astronomical detail with modern sequencing equipment. My advisor was Diana Nemergut, and the instant I met her all doubt whether or not I should do a postdoc vanished - we talked about microbiomes and epidemiology, about the continuum between microbes that are “good” vs “bad” for the host, and I moved to Durham ecstatic for years of exhilarating research.
Tragically, Diana was diagnosed with cancer and lost her battle to cancer just a few months into my postdoc. While Diana was going through chemotherapy and winning her age bracket in 5Ks, she worked with the biology department set aside her startup funds to allow her postdocs to continue their research. My academic career was uncertain, and my wife & I had medical bills to pay for her shoulder surgey, so I started moonlighting as a statistical consultant for a quantative hedge fund, bringing tools, tricks & instincts from my PhD work to the fascinating world of finance. While a postdoc, I developed statistical tools to study ecological patterns like which lineages of microbes are associated with disease or how to incorporate our knowledge of evolution into the ways we analyze large ecological datasets. When I was not a postdoc at Duke, I was a quant at a low-beta hedge fund tearing through dozens of datasets & terrabytes of data, systematically studying financial systems and developing statistical tools to analyze alternative datasets and forecast the returns of equities across a range of stock markets worldwide. My interests stayed weird.
I left Duke in 2017 to start second postdoctoral position in Bozeman with Raina Plowright studying pathogen spillover from wildlife to people. With Raina and her incredible global community of experts, I was modeling, analyzing data, and forecasting pathogen spillover from bats to people, feeling a bit more like my two lives had merged. I also negotiated a consulting agreement with a group at EPFL in Switzerland, helping their group study microbes associated with glacier fed streams and learn what we’ll lose as glaciers recede from climate change. So, I was a glacier-fed stream microbiome guy in the mornings (afternoons in Lausanne), an epidemiologist/ecologist postdoc by day, and a financial data analyst by night. When COVID hit, my weirdly interdisciplinary background put me on my unusual path.
As global financial markets tried to make sense of COVID, I was doing proper epidemiology - reading case reports to understand the clinical presentation, reading papers’ supplemental figures for details like PCR test performance, analyzing the data for myself, and diving into the latest models with sharp attention to the detials. I was also helping clients in the finanical sector make sense of this oncoming crisis. I was sitting on calls in which world leading epidemiologists told the world’s largest investment bankers what was coming. On the calls, I evaluated the epidemiology presented and tried to infer the beliefs of major investment banks and economic thinkers as the sought to translate “COVID” into thinks like “consumer spending”, and “risk of default on small business loans”. In academic epidemiology, I was relatively unknown, yet in finance I sat in on major calls with a speaker to my hearing aid, fully able to understand what epidemiologists were telling international managers identifying what was either presented inaccurately or lost in translation. Arbitrage awaits in the shadows of overconfident, false beliefs.
As surely as someone fluent in two languages can spot a gap in translation when some word has multiple meanings or some important cultural connotation is missed, I was able to identify the gaps between what was said and what was meant, the unstated uncertainties, the datasets even the consulting epidemiologists - the most famous epidemiologists in the world - had evidently not analyzed as thoroughly, supplemental figures they had not looked at, or important aspects of clinical reports like PCR test false-negative rates that they were not aware of.
I was studying COVID from the first day we heard about it and deploying an unusual interdisciplinary set of tools to study COVID, forecast outbreak trajectories, and make assessments about popular beliefs and misconceptions of COVID outbreak scenarios. While the bulk of mathematical epidemiology fits curves to historical datasets, the only work worth doing in finance predicts out-of-sample data. The years I spent devouring those alternative datasets to forecast returns across global stock markets prepared me for COVID. As an epidemiologist, I uncovered findings about COVID that differed from the science du jour, findings that were able to predict seemingly anomalous characteristics of out-of-sample outbreaks. As these findings were released and as my predictions came true, I was swept into management consultations, political battles, and scientific disagreements at the heart of the pandemic.
From my perspective as an epidemiologist, COVID was tragically fascinating. I studied morbid time-series of outpatient visits, hospitalizations, and deaths. I published cutting-edge research and entered the mosh pit of epidemiologists on Twitter, revealing tragic challenges with the scientist-scientist, scientist-public, and scientist-policymaker interfaces. I got to know a world of brilliant epidemiologists, yet I also felt I burned my bridge to an academic career by speaking my truths to world-leading epidemiologists on Twitter whose theories or policies I disagreed with. While I’ve been fascinated in epidemiology since my undergrad in 2007, there was much love lost during COVID.
From my perspective as a financial data analyst, COVID was revolutionary. In finance, the “efficient market hypothesis” that many love but few believe saw complete rejection in my eyes. The efficient market hypothesis posits that publicly available information is priced in, so that things like the number of deaths from COVID and all possible models and theories of COVID outcomes would already be efficiently factored in and nobody could have a better theory that beats the market. If there were something to know - like a pandemic will explode rapidly in March in NYC when the world’s experts say cases will grow slowly & curves flatten to peaks around June/July - such things-to-know would be known-already, believed in proportion to some rational posterior probability, reflected with Patonic pefection in ever day’s asset prices, and so there’s no way to make a risk-free profit. Under the Efficient Market Hypothesis, all is known and believed about the natural world and its economic subsystems, and any new information or even theoretical advancement would instantaneously diffuse throughout the world in wonderous efficiency.
The efficient market hypothesis is dead. COVID revealed global information-sharing processes from epidemiology to finance by which the bulk of the world’s investment volume thought they knew what was happening - they believed what people told them on the call - but the things they didn’t hear on the call, the figures the epidemiologist hadn’t scanned, and the theories that couldn’t penetrate the noise on Twitter all piled up to create mountainous market inefficiencies. I sat on calls with epidemiologists telling economists that the train of cases would arrive slowly & in an orderly fashion, yet I discovered the oncoming bullet train a pandemic. I tried to warn others about the bullet train but encountered threatening signs that speaking up would lead to punishment. So, I turned back to the economic systems and traded the arbitrage opportunity to generate extraordinary (and, in my eyes, risk-free or improperly risk-adjusted) returns. Unable to share my findings in science without fears of political repercussions, I “put my money where my math is”, albeit with the low volume one can expect from a postdoc with a weird employment setup.
Most people at the time of this writing do not know there are “two theories” of what happened in COVID. Most epidemiologists haven’t heard of it, much less heard its full defense. Even writing “I have a theory,” I can hear several readers rolling their eyes. Such is the challenge of injecting new information in our scientific system: during COVID, there was an extreme, vigilant, and well-intended effort to prevent “misinformation”, and my own theory was caught in the crossfire. The world of epidemiologists became hostile to scientific novelties like Michael Levitt’s Gompertz curves at exactly the time when we needed novelties to learn about this novel virus. My theory was a novelty and, like Levitt (a nobel laureate), I was subject to hostilities yet I was not a nobel laureate so I couldn’t handle the pressure. I left my postdoc, but after repeatedly corroborating my own predictive theory of COVID outbreak patterns & history and its large network of immunological, virological, and epidemiological implications, I finally put literally every penny I owned & had earned throughout the pandemic into a margin trade the day we heard about Omicron. I won big, I guess…
Financial winnings from being a scientific outcast who was right are both fascinating disproof of the efficient market hypothesis and sources of pain, proof of an imperfect world. The market inefficiencies were the result of poor information diffusion, itself the result of inequitable social processes in science - homophily (loving similar views), hostility towards novel views, false consensuses, overconfidence, and incumbent scientists and their paradigms, holding positions of real power and both willin g& able to suppress minority views or valid alternative theories. I thought these social barriers to scientific progress were a relic from the Phlogiston era and that the Feudal hierarchy of academic epidemiology would flatten in a crisis, that the right answer would bubble up quickly and efficiently so the whole world would be informed of the cutting-edge of science as theories bubble and pop. Instead, these socioscientific inefficiencies became institutionalized by state-of-emergency powers, by editors of major journals picking sides (namely, the sides of their old-time friends at the top of the Feudal system), by media outlets choosing who’s “an epidemiologist” and who is not, by federal decisions that some novel science (like vaccine evasion pre-Provincetown) is “misinformation”, thereby dramatically limiting the ability of our world of scientists to discuss, debate, and deliberate for more rapid and equitable advancement of science. Conventional models were wrong and my unconventional models, methods & findings were misinformation.
I say “the models were wrong” not as someone who flipped a coin at the NYSE and wants to rewrite the history of their lucky trade, but as an epidemiological modeler during market inefficiencies driven by gaps between what people expected from COVID and what actually happened, gaps that could be closed by a superior understanding of the natural epidemiological process engulfing the globe. I’d like to qualify my knowledge in epidemiology to someone outside of epidemiology (as those in epidemiology can tell from my dialect that I speak their tongue). I hate the word “expert” as it creates a dialectical contrast of non-experts; while knowledge is real, the social construct of “expert” is a Freirean trap that lures people privileged with formal education to close their minds to the wisdom of “non-experts”. “Expert”, and the credentialism used to reinforce its power, is a form of discrimination that can be readily deployed to cast doubt on anyone, including young scientists, interdisciplinary travelers, and people with critical knowledge in other fields. Let me just qualify my knowledge of models without saying the word “expert” and instead saying: I am both a mathematician and a biologist. I can read any mathematical epidemiology & COVID forecasting paper, understand not only their math/stats methods but also the laboratory techniques used to estimate their critical parameters, I’m able to identify limitations of both mathematical & (to a slightly lesser extent) laboratory studies. I can identify critical lines of future work. I’m infinitely curious about the world and self-identify as an lifelong learner. To me, expertise, if it infects one’s head, closes one off to the massive universe of unknowns. I’d rather chase the incredible universe of unknowns as an epistemic hobo than confine myself to a miserable, pitiful shack of what I think I know.
I know things, and I knew the models were wrong. I also want to note that I use the word “wrong” in describing forecasts not to insinuate malintent in the hearts of scientists who proposed them. All models are wrong, some are useful. The vast majority of previous theories about the natural world have been rejected, yet they served their purpose as stepping-stones on our journey to understand the universe. We have every reason to believe that many of our current theories will be overturned. That’s the beautiful, evolving, socio-epistemological system of science. Our goal a scientists is to become ever less-wrong, more-useful. I have no beef with modelers, but I do falsify models and detest inefficiencies in the global social processes by which we evaluate models, theories & more. At many stages of the pandemic, we had more useful models available that would’ve made the world less surprised about what was to come, but those models couldn’t penetrate the discussion. The models that took up the oxygen in the room did so not because of their accuracy but because of the usual reasons why science can be so slow to adapt - they came from famous people and famous institutions, from the incumbent teams who held an oligopoly on scientific discussions, who were long time friends of editors at major journals… at the exclusion of a much larger world of scientists rendered relatively voiceless in the face of institutional & social-media megaphones.
Throughout the pandemic, I continued to watch the data, to study, model, and forecast outbreak characteristics and provide these insights to managers. Now, 2.5 years after I first started COVID, I’m more convinced than ever that the world’s common understanding of COVID is wrong. The world’s current, and I believe incorrect, theoretical path started from the earliest models, the inefficient marketplace of ideas around those models, and the path-dependent social, political, and scientific course we took from early 2020. Throughout the pandemic, I set up hypothetico-deductive dashboards to test these incumbent models against my own, and I saw these incumbent models fail everywhere they were tested. Those same models justified policies that predictably caused immense collateral damage concentrated in the poorest people in the world. Travel/trade restrictions and lockdowns worsened famine in people outside our borders. School closures caused one of the most inequitable disruptions in childhood educational attainment in recorded history. Models informed policies and policies hurt people.
My history of COVID tortures me as the predictive accuracy and out-of-sample insights of my theory, which I would imagine are cause for celebration, accolades and awards, have proven insignificant against the insurmountable power granted to incumbent scientists with ties to major media outlets capable of driving narratives, manufacturing consent during a global crisis, and winning scientific battles not by sharper minds and better predictive theories with fewer anomalies, but by their ability to write articles in the New York Times or the Atlantic, by their weekly presence on BBC, by false and arrogant claims of scientific “consensus” in dominant peer-reviewed journals, by having more followers on Twitter, and by galvanizing mobs of people and normalizing hostilities towards fellow scientists to cause minority scientific theories and novelties, however right they may be, to face unbearable obstacles to fair consideration in the marketplace of ideas.
While COVID was no fun, and while there’s considerable pain in my story, I don’t want you all feeling sorry for me. My theory helped me make a small fortune. I learned more in 2.5 years than I expected to learn in a decade. Now, I’m deploying my capital gains and knowledge to do the greatest good I can for the world in light of what I know now. I’m an optimist and a believer, I live a passionate and full human life and I see a path forward. Amidst the travails and tragedy of COVID, there was levity, there were memes, friends, and joyful moments. I couldn’t overcome my infinite sadness of failing to help the world chart a better course that reduced all-cause mortality & morbidity. However, even if just for my own mental health, I had some fun. Below was one such funny moment, a portrait from my weird COVID journey.
I present this honest account of the “other” theory of COVID-19, which I call the “predictive” theory. This theory was conceived and pursued by a mathematical biologist who studied epidemiology since his undergrad thesis in 2009, and who worked with hedge funds since 2016 learning how to predict things. The theory predicted many things that were unpredictable or anomalous under the conventional theory and history of the COVID-19 pandemic. This theory was not presented on most calls to most managers, yet it produced accurate predictions on critical things like “how many ventilators will NYC need, and by when”. This theory produced inconvenient truths about the probable vaccine-evasion of Delta, a truth that would get people banned from Twitter at the time. Finding this “other” theory - and failing to reject it in every outbreak I analyzed - made it impossible for me to believe the conventional theory and narrative of the news, yet COVID-19 was not a safe time to be unconventional, certainly not if you lacked the power of tenure & distinguished professorships. My discoverying this theory, my inability to disprove it, my repeated disproofs of the conventional theory, and the inhospitality of vocal influencers in epidemiology, all led me to leave my academic career track, so I may never be a professor who teaches this theory to his students. I present the theory, and my story, for posterity and closure. Like all theories, I know this theory is incomplete and there is more to learn, and, rest assured, I am still studying this - being in front of the news is way more fun than reading the news.
I hope that by providing my own account, with all the pieces of the puzzle in place, with a vantage point of time over this contemporary coliseum of COVID science and policy in which I have been a mere gladiator slashing in the melee, tomorrow’s historians may see something we don’t see today. Something about science, epidemiology, financial markets, social & information ecosystems, and a pandemic playing out with a bunch of hominids slinging shit on Twitter, something that improves the ability of science to help society during a global crisis.
The beginning: Spring 2020
I differed from conventional models & common narratives starting from the case growth rates in Wuhan between January and February 2020. Looking at the same data but using different methods, conventional teams estimated cases grew slower, I estimated cases grew faster. Slower case growth rates meant fewer unascertained cases and a higher risk of death per case, whereas faster case growth meant more cases and fewer deaths per case. Slower case growth meant we were ascertaining most of the cases, could test-trace-isolate (TTI) to snuff out local outbreaks at a continental scale, and wouldn’t see major US outbreaks until July 2020. Faster case growth meant we were missing most of the cases, that TTI would fail, that massive surges could hit New York as early as March 2020. Scientific differences in the estimate of a parameter - how fast cases grow - led to vastly different prior beliefs about pandemic burden and policy efficacy.
I’ve already shared some of my story on COVID-19 on how I estimated a much faster growth of cases in February 2020 that conflicted with estimates publicized by the most famous epidemiologists in the world. To be more specific, major institutional teams saw 6.2 day doubling times with June/July 2020 peaks whereas I saw 2-3 day doubling times with a high probability of a fast and massive March 2020 surge in places like NYC, and I saw this by mid-February 2020. I tried to get my findings into the right hands, only to encounter a toxic mix of credentialism, elitism, and arrogance - how could a weird, interdisciplinary guy in Montana possibly produce better forecasts on a GLOBAL PANDEMIC than credentialed infectious disease epidemiologists at Harvard, Oxford, or the Imperial College London?
I backed away in disgust from what I perceived to be elitist academic research cartels and just shorted the stock market in mid-February. It was cool to predict a black swan event and I made a lot of money. I also felt like complete crap that I couldn’t share my findings to a curious and kind scientific community. When I looked at epidemiology as a “community”, I saw a pack of wolves with fangs, gangs with knives looking to stab someone or at least quote-tweet-call-out their rivals. I saw hostility and persecution from the online scientific community. I wrote more on the informal social control in science, the culture of hostility inventing crimes and enforcing punishment for scientists that deviated from a mainstream view presented by incumbent experts.
My article on “The Science Not Shared” connects my perception of professional risks from sharing science to the online hostilities directed at Ioannidis, Levitt, the Great Barrington Declaration signers & dear friends Jay Bhattacharya, Martin Kulldorf, and Sunetra Gupta, and more. I will defend these scientists until my last breath - they are sincere, good people. Both their science & their policy proposals were shared with the world with good faith, compelling scientific reasoning, and unique personal & scientific perspectives. These scientists all have a common thread: the deviated from the narrative. Ioannidis said it’s possible the epidemic isn’t as bad as slow-growth models suggested & it’s possible our policies could cause great hram. Levitt used unconventional methods to observe & utilize a clear, regular deceleration of case growth rates. Gupta started off observing, with Jose Lourenco, that fast case growth in the UK introduced great uncertainty about how bad the outbreak would be. At this time, Ioannidis, Gupta, Louranco, Levitt and I were all converging on the same reasoned, evidence-based disbelief of conventional models, their policy implications, and the narrative told to policymakers, the international financial system, and every mainstream media outlet.
I often call the dominant view “The Narrative.” I realize this, especially from a dude in Montana, can sound rather conspiratorial. So, please allow me to me elaborate. “The narrative” was an evolving, amorphous but narrow belief system combining scientific, political, economic, and moral values & beliefs. The scientific observation of June/July peaks was correlated with the political belief that masks shouldn’t be recommended, which later changed to a political belief that masks should be mandated; an unjustified scientific belief that SARS-CoV-2 was indisputably the result of a zoonotic spillover directly from a bat or pangolin was strongly correlated with a fierce intolerance of the Great Barrington Declaration, its signers, and anyone (like me) seen to be guilty by association and endorsement. The Narrative could not be changed by anyone - there were only a few ‘high priests’ (read: “experts”) who were allowed to authoritatively change this belief system. Fauci, Birx, the WHO, and Twitter-famous Epidemiologists could change The Narrative. They had institutional power that gave them quick access to editors of peer-reviewed journals so they could publish their opinions masqueraded as scientific authority in accredited journals; they had massive Twitter followings, connections to editors in all mainstream media outlets, and more. The Narrative was rarely inclusive of diverse perspectives, rarely open to deliberation or discussion by non-”experts”, and secured itself by weaponizing institutional advantage of the priests to win polical and scientific battles. The Narrative was conceived at the top by incumbent experts and disseminated to the bottom by their media, with little room for feedback. As Euthyphro told Socrates that “piety” is whatever the all the Gods believed was pious, what was pious in “The Narrative” was what the all the priests of COVID epidemiology believed. Deviation from The Narrative was impious, and we saw the consequences of impiety from Ioannidis to the GBD.
There are always differences of opinion among scientists - there is nothing “all the Gods” agree on if we’re being broadly inclusive of “all scientists”. This doesn’t mean there isn’t truth or that the process of science isn’t good at helping us find it, it just means that we need to have humility about our current scientific beliefs, create space for disagreements, and avoid posturing about a false consensus as if spiking a football at the 40 yard line. These scientific virtues were all the more important as we raced to understand a novel phenomenon like SARS-CoV-2. All of us scientists are different blind people feeling different parts of the elephant of nature, and while The Narrative felt the trunk, those feeling the tail were bad, wrong. They weren’t just incorrect, they were anti-science purveyors of misinformation, with conspiratorial ties to hidden, dark buckets of money. It didn’t matter if our observations, predictions, or proposals were vindicated with time - the scarlet A of Antiscience stuck with us.
We rely on scientists to help the world understand our universe, and we give scientists the unique power & privilege to consult the world in times of crisis. Yet, scientists work in an complex, hierarchical, inequitable, and inefficient social system, and this social system impacted the interface between scientists and society. Scientists formed a very active and very aggressive bubble on social media and they weaponized credentials, institutional powers, editorial connections, and media connections to win debates. Scientists were cogs in a global scientific system of political and economic incluence and dominated discussion so completely it feels as if they became a mob with no checks and balances, authoritarians who decide information from misinformation, and this unchecked power reinforced an open hostility towards peers and unscientific credulity of whatever the priests said. The nebulous ideas they so fiercely and blindly protected - The Narrative - became an unscientific system of belief with the unique social, media, and even governmental power to crush dissent and exclude diversity. This mob impacted my own attitudes and actions during the pandemic, including my reluctance to speak up when my findings disagreed with the mob’s beliefs du jour.
To summarize: I stared at findings contrary to conventional belief, I tried to share them only to find unconventional hostility, a pattern of discrediting me as “not-an-epidemiologist”, “just-a-postdoc”, “not-from-Harvard”, or “non-expert”. I experienced firsthand the extraordinary inequalities and hierarchies of powers in academia, such as one professor with direct access to the head of DARPA’s Biological Technologies Office (which funded my Montana postdoc) called up my PI’s program manager’s boss (big boss man) and triggered very threatening institutional feedback from “upstairs” that encouraged me to cease & desist or else I worried I could lose my job or impact the reputation of people I worked with in DARPA. I retreated from scientific information-sharing, shorted the market, and made a small fortune as my forecasts came true.
Here’s a picture of me in the Empire State Building in late February when the S&P500 dropped. The excitement of predicting a Black Swan was exhillarating, a tale for the ages as previously unimaginable sums of money showed up on a screen thanks to an epidemiological theory translated into financial forecasts. The next morning, as I sat in the office drinking coffee & looking at the vibrant city below, I saw a world of people like the ones I grew up with in Albuquerque’s public schools, hustling in the streets, subways & buildings behind me, and this world of people was completely unprepared because I couldn’t get the message and the models out to them.
The first forecast that turned a mob against me and motivated shorting the market ultimately came true. Goaded by the torment of the COVID Short, I forced myself back into epidemiology to share this work. On March 5th, I called Eric Alm, a colleague from the microbiome world who I knew was working with Biobot Analytics on wastewater surveillance of opiods. I recommended Eric et al. look in Boston’s sewers for SARS-CoV-2. Eric is a beautifully authentic and kind scientist, one of my all-time favorite people in science, so he seemed like a good place to start making calls. After hearing the theory, Eric mentioned that he thought I was crazy, but the good kind of crazy, and he agreed to look.
At the time, wastewater surveillance for SARS-CoV-2 was not a thing. Researchers were actively learning about which tissues the virus infects, from which tissues it can be cultured, and more - fecal shedding was a demonstrated but not widely appreciated observatoin of SARS-CoV-2 virology, epidemiology, and host physiology. The Spike protein of SARS-CoV-2 binds ACE2 breceptors, ACE2 receptors are found along the entire GI tract, stools of pediatric patients had been documented as testing positive, and the prominence of common sites of fecal/oral transmission - nursing homes, cruise ships, hospitals - all generated the hypothesis that we should look at poop. If there’s something you should know about microbiome people, it’s that they love to look at poop.
After Eric, I toughed my skin & started broader outreach to my network of colleagues, calling in favors to get connected with epidemiological forecasters at the CDC. My early March outreach yielded the opportunity to share my observations on a CDC forecasting call on March 9th. On the CDC call, I gave my case of fast growth and extreme uncertainty of burden, with a high probability of lower burden and an oncoming, explosive March 2020 surge in New York City. We discussed details, I tried to emphasize the “uncertainty” part but got sucked into explaining how this could be consistent with the observations from the Diamond Princes cruise ship in which nearly a dozen people had died. I talked about PCR test false-negative rates, the age-distribution of IFRs and cruise ships. I showed how this is consistent with the Imperial College Traveler-Screening survey. At the end of the call, the world-renowned epidemiologist leading the call said “I don’t believe it” and then promptly ended the call.
Just 9 days after the CDC call, medical managers in New York saw my predicted 2-day doubling times in ICU arrivals across providers in New York City. We got connected and they roped me into management circles during the worst US outbreak of the entire pandemic. In mid to late March 2020, my theory suggested immense uncertainty about how high the peak of this surge would be. ICU arrivals of patients on ventilators are doubling every 2 days, the entire pandemic plan built on slow growth & 6.2 day doubling times went ou the window. Italy locked down around the time of the CDC call, large international hubs all over the world were locking down. New York City locked down. How many people were going to die? How many ventilators does New York need? What should we do?
I worked with a crew of close colleagues who I love dearly, would go to the end of the earth for, and fear naming as I’m scared of potential professional guilt-by-association with me. We engaged with the NY State COVID task force by providing forecasts of medical demand. The dominant institutional models with 6.2 day doubling times shifted their doubling times in light of new information, but they did not shift their case-severity proportionately and so these models estimated 40,000 ventilators would be needed at the peak of the NYC outbreak. I was uncertain about severity but saw decelerating growth rates of ICU admissions and used Levitt-style projections of decelerating growth rates to estimate only 11,000 ventilators would be needed. The peak ventilator demand was ~10,800, if I recall correctly. Cuomo subsequently ranted against modelers’ estimates being “all wrong”. Of course, that missed an important historical note that there was, in fact, one model that was right on both the timing and the unconventional method for estimating the magnitude of the NYC peak.
The reason that model didn’t get heard or popularized was because its primary author was subjected, starting in February 2020 and continuing throughout the entire pandemic, to a torrent of attacks for the model’s implications. I never received criticisms of the model itself, only that the implied lower-severity was “minizing COVID” and “disrupting the public health message”. My impieties included contextualizing COVID within the range of other common causes of death - called “trivializing deaths”. The social media scene of COVID was a pretty harsh workplace environment, maybe not the best communication platform to accelerate science.
When we published the observed surge of patients visiting doctors with influenza-like illness (ILI) in March 2020 and its implied (slightly!) lower severity than estimated by the Imperial College London, a long-ish time colleauge and ecology assistant professor from Georgetown quote-tweet-dragged me over the coals of followers in a digital pillory, saying I disrupted the public health message and could be responsible for the deaths of millions. Professors with large Twitter followings and intense personal views normalized scientist-on-scientist attacks with morally Wagnerian accusations of impiety - disrupting the message, responsible for millions of deaths. This behavior normalized by being done without retribution and the way people seemed to pile-on to call-outs. You could palpably smell the crowd getting more intolerant by the tweet.
Mobs suck.
On a funnier note from early-mid 2020, the ILI paper led to an interview on WebMD in mid-April. I jumped on the call sleep-deprived, overcaffeinated, morally conflicted, mobbed, and I hadn’t had a haircut since before the NYC trip in February. I still chuckle to myself about being the guy from Montana who was on WebMD looking like Grizzly Adams, the Unabomber, and/or an alcoholic at his first AA meeting. My wife had told me beforehand to trim my beard but I didn’t think it would be that bad. I relearned an important lesson: my wife is always right.
Why the Imperial College Report 9 was wrong
This section is going to take a deep-dive into methods and observations leading up to the NYC surge. Specifically, I want to explain why I didn’t believe the Imperial College London model and all other conventional teams’ models derived from it. Feel free to skip this entire more boring, technical section without skipping a beat on the bigger story. My main objective in this section is to articulate the exact ways I knew - at the time & not from my armchair in the future - that the Imperial College report and conventional models would underestimate case growth rates and overestimate pandemic burden.
The IC report 9 was pivotal because it estimated an unmitigated COVID-19 outbreak could kill millions (plural) in the US. Specifically, they estimated 2-3 million could die in an unmitigated outbreak. The estimates of burden ultimately come from estimates of three other quantities: the case growth rate, the infection fatality rate, and the serial interval distribution. I had no disagreement with the methods used to estimate the serial intervial distribution - that literature was sound & all signs pointed to agreement. I disagreed with the estimates of the case growth rate and the infection fatality rate. Also, I knew that, because of how we estimate the infection fatality rate early in the pandemic (namely, dividing observed deaths by simulated infections), these two quantities were dependent upon one-another - faster case growth rates lead to more simulated infections & lower infection fatality rates. To say the same thing differently, we all trusted the observed deaths, but a faster case growth rate from the same pandemic starting in late November 2019 would generate more cases. More infections, the same number of deaths —> lower infection fatality rate. One last time: due to limited data & the way we estimated model parameters early in the pandemic, faster growth rate ==> lower infection fatality rate.
The IC report 9 used two papers to estimate both the growth rate and the infection fatality rate. One paper was based purely off of case doubling times in the Wuhan outbreak: they estimated a 5-day doubling time for China’s outbreak starting December 1, 2019. Their estimates were wrong, however, because they included many dates over which the exponential growth rate was not constant. Dr. Michael Levitt and I independently observed that the growth rate in China was not constant, but rather it was decaying (Levitt used a Gompertz curve to fit the growth rate trajectory).
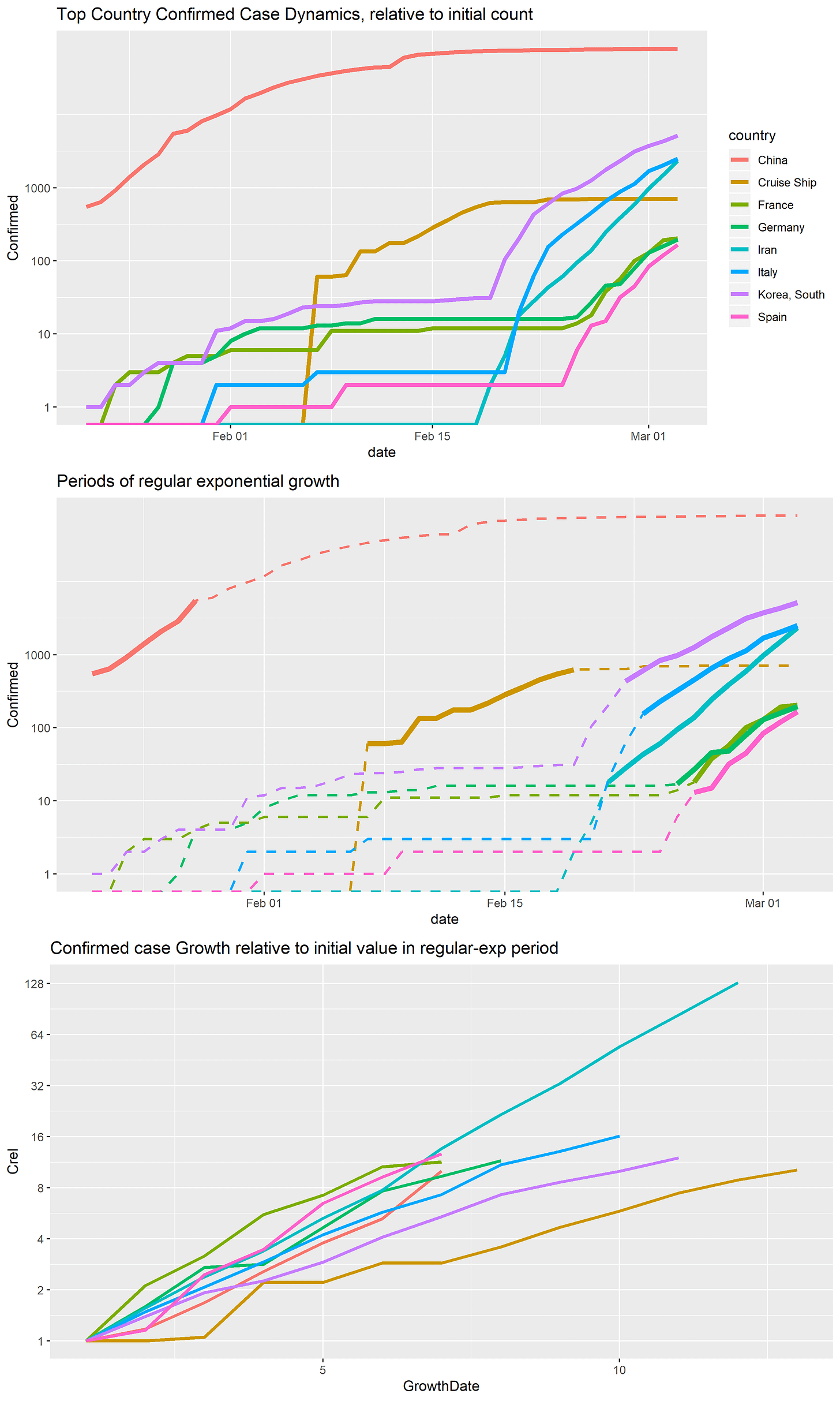
If growth rates were constant at their initial fast rate and then they declined over time, fitting a single growth rate over this whole time series will give you a growth rate of 5-6.2 day doubling times. Fitting a growth rate to the initial period of exponential growth gives you 2-3 day doubling times. If you started an outbreak in China on December 1, 2019 and cases doubled every 2 days, then in just 60 days you could infect over 1 billion people. If cases double every 3 days then in those same 60 days we’d expect 1 million people infected. If they doubled every 6 days then we’d expect 1,000 people infected with wide error-bars given the superspreading nature of SARS-CoV-2. This, of course, is why John Ioannidis cautioned about the massive uncertainty, and why Jose Lourenco, Sunetra Gupta et al. called for serosurveys in the UK in March 2020. Growth rates used by the Imperial College Report 9 were too slow, their infection fatality rates too high, and the fast growth rates made most of our uncertainty of pandemic burden come from an unknown date in November-December 2019 when stuttering transmission chains got a foothold and cases started growing exponentially, doubling every 2-3 days.
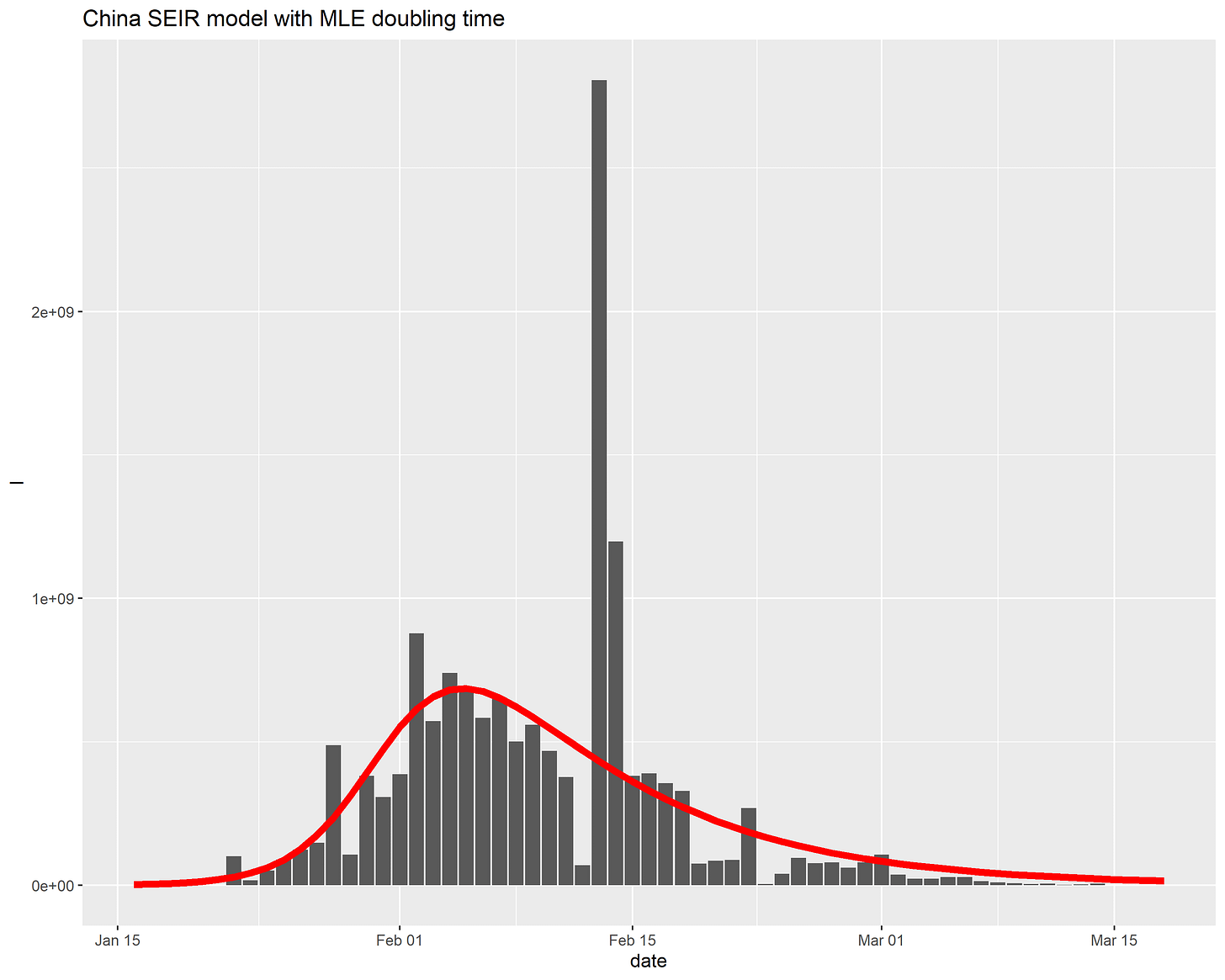
The other paper used by the IC report 9 to estimate that 3 million people in the US could die relied on traveler screening. In their report 7, the same Imperial College team swabbed the noses of travelers. Most travelers were only swabbed once, and a negative test was assumed to mean the repatriated traveler did not have COVID. However, sometimes tests are wrong. From the temperature & handling procedure to primer batches and only swabbing one nostril instead of two, many factors can make a test more likely to be wrong. None of these factors - let alone the entire idea that a test could produce a false-negative result - were used in the IC report 9 when estimating infection fatality rate from traveler screening. In the early pandemic, patients who were hospitalized and clearly infected would be swabbed repeatedly, and nearly 50% of those swabs would come back negative. That was in a hospitalized patient, and patients with severe symptoms might reasonably have higher viral titers and test positive at a higher rate than the asymptomatic or subclinical patients (I’m not saying that was true, just that we couldn’t rule it out). So, the 50% false-negative rate PCR tests of hospitalized patients, applied to the early, rushed swabs of air travelers may underestimate the false-negative rate of the traveler screening study. As the false negative rate goes up, the prevalence we should’ve used for repatriated travelers goes up. With a higher prevalence, there are more cases and, consequenty, a lower infection fatality rate.
Those are the reasons why I disagreed with the Imperial College report 9 and its estimates of “millions” of US deaths in an unmitigated pandemic. Between 2-day doubling times yielding 1 billion cases and 3-day doubling times yielding 1 million cases - a 1,000x difference in the implied infection fatality rate - I was uncertain and felt growth rates, lacking a clear start-date, were an unstable basis for estimating infection fatality rate (these are almost the exact arguments of Lourenco, Gupta et al’s 2020 paper studying the UK outbreak). The repatriated traveler survey was complicated by the vastly different methods used across regions and the failure to account for a likely high false-negative rate for swabs of just one nasopharynx in busy travelers trying to get through customs.
Infection fatality rates, derived indirectly from case growth rates & from repatriated traveler surveys, determined model estimates that millions in the US would die in an unmitigated outbreak. These models anchored the beliefs of many epidemiologists that COVID would, in fact, kill 2-3 million or more in the US if unmitigated. However, I read not only the reports but their supplemental files and the citations behind these critical model assumptions, and, in conclusion, I felt these models were wrong and biased towards overestimating pandemic burden. Growth rates estimated were too slow because growth rates were not stationary. Infection fatality rates were too high because they didn’t account for false-negative rates of cruise ship passengers (or the comorbidities of Diamond Cruise ship passengers and whether or not those are representative of the infections in an unmitigated US outbreak).
John Ioannidis, a meta-scientist familiar with how peer-reviewed papers are not immune to being wrong, was the first person I noticed who said the quiet part loud, that the emperor had no clothes, that we’re making decisions without reliable data. I watched in horror fellow academics swarmed after Ioannidis like online africanized honeybees with a license to kill. I agreed with the observatoins of Levitt who was charged with publicizing “lethal nonsense”, and I agreed with the core claims made by Ioannidis (a distinguished prof at Stanford) who became a pariah among academics on Twitter. I saw them both get swarmed with hostility that outlasted either of their active participation in the discussion - so many people hated COVID dissidents with a passion. They believed we were immoral and corrupt and justified & normalized online pile-ons, deplatforming, and other efforts aimed to exclude scientists from science. Seeing what I saw, what would happen if I, a postdoc from Montana State University, shared my work? What happened in public against Ioannidis and Levitt is the same cultural autoimmunity I encountered in private and in public when trying to disseminate my findings. It was a culture of not just homophily - preferring one’s own views, as is common in science - but outright allelopathy, or trying to kill or suppress opposing views. The social mechanisms used to kill opposing views were not hypothesis-testing to disprove proposed hypotheses nor rigorous examinations of mathematics and methods to find flaws. There was a new moral code arising with an unwritten code of conduct and punishments for violation and little compassion or mercy or benefit of the doubt granted to deviants. This cultural autoimmunity occured in the hierarchical academy, on Twitter, in mainstream media outlets, conferences, scientific journals, and other institutions converted into weapons of war in scientists’ competition for narrative power.
One example of this was when people like Carl Bergstrom and Gavin Yamey, in late 2021, accused John Ioannidis of a research ethics violation for using Twitter handles in John’s paper investigating the citation records of Twitter scientists driving the scientific narrative. John was saying: the people calling themselves experts on Twitter aren’t necessarily the experts, and that made famous people on Twitter very sadmad. Carl, Gavin and others in the mob called for retractions and professional consequences for Ioannidis for not consulting an Institutional Review Board (IRB). However, Twitter data is public and no such rule exists that you have to have IRB approval to publish handles of Twitter data. IRB experts agreed that Ioannidis committed no wrong. This situation was ironic because previous Twitter studies had been published with the goal of discrediting what they called “anti-mask” groups on Twitter. Those studies published Twitter handles and they were not called-out, because those studies were reinforcing the narrative and saying people like me are anti-mask (as I was solidly within the part of the network they *ahem* impartially labelled “Anti-Mask”). After all the studies of Twitter data in this time period, many of which publish handles, there was but a single study from Ioannidis, and people wanted to sink him for it. I objected strongly to the behavior of Carl, Gavin et al. I argued that their selective ethics was not ethics - it was, rather, a good example of the targeted hostility and abuse of institutional powers, trying to weaponize research-ethics to create new tools to call-out Ioannidis in a transparently intolerant effort to exclude a scientist who deviated from The Narrative. Simply pointing this out led to me being blocked by Gavin Yamey, Carl Bergstrom (Carl called me “insufferable”). Similar exchanges - whether sharing my findings, speaking my truth, or trying to stop the mobbing - got me blocked by many other powerful people in the field.
I had a model that differed from conventional models with the same seriousness as Levitt and Ioannidis, but I also had far less protection of tenure, Nobel prizes, or distinguished professorships. I was worried about the clear and extreme online hostility. I experienced it first-hand and continuously throughout COVID. The cultural autoimmunity of the mob was a pathetic sideshow compared to the math, methods, and model performance that we scientists should’ve talked about. Behind the outrage and moralization, there was a critical unsung, legitimate scientific disagreement about mathematics & methods. Our disagreements in early 2020 determined the path-dependent course of history of the COVID-19 pandemic.
Undaunted by the warzone of Twitter, the pandemic continued to wreak havoc with the regularity of the natural system that it was. To recap: as I’d predicted, the New York City outbreak saw 2-day doubling times of ICU arrivals from March 15-18, and I was roped in. I looked at the data of ICU arrivals across providers in New York and immediately saw the same decelerating growth rates that Levitt and I saw in Wuhan and were unlikely to have been caused by interventions (as the lockdown was, by that time, not yet implemented). I used the same concept of Levitt’s methods (projecting the early and continuous deceleration of growth-rates) to estimate 11,000 ventilators at peak demand. Influenza-like illness arrivals in NYC peaked on March 27th - the exact date I’d predicted a peak back in early March - and ventilator demand crested at ~10,800.
Seeing my models’ and methods’ successful predictions led me to seriously consider the hypothesis that the New York City outbreak had actually depleted its susceptible population. If true, it would suggest that future US COVID outbreaks are likely to see as much or fewer deaths per-capita due to COVID than NYC saw on March-April 2020. This hypothesis was presented to the NY State COVID Task Force.
Mask-wearing
The McKinsey team working for Governor Cuomo heard that hypothesis, acknowledged that I had some good predictions but it was still possible that my model and its hypothesis is wrong. They articulated their policy crux: they locked down New York and, while our forecasts were strangely accurate, there was still the possibility we were wrong. What if they reopened, cases rose, and people died? On the call, I pointed to Japan. While most other outbreaks at that time saw 2-3 day doubling time, Japan saw 7-9 day doubling times suggesting much lower transmission. Japan’s policy was a mix of educating people about how transmission happens and encouraging people to use masks. Japan’s early success at reducing transmission suggested it may be possible to reduce transmission and mitigate the burden from COVID-19 outbreaks without locking down. Despite Anthony Fauci and the CDC not recommending masks at that time, I recommended masks, along with a platform to recruit Independents & moderate Republicans to the reasonably justified, precautionary mask-wearing experiment.
I said one could encourage people in the center & right to wear masks by saying things like “when I wear my masks, it says I love my neighbor; when I wear my masks, it keeps the doors open for NY businesses; when I wear my masks, it keeps hospital beds available for others who need them.” Cuomo went on TV to encourage mask wearing a few days to encourage mask-wearing in New York and used many of those lines. I wrote them again for my own community of Bozeman, Montana. I wasn’t the only person who recommended masks at this critical juncture but I was ironically referred to as “anti-mask” and “COVID denialist” or “COVID minimizer” (again, in the MIT paper about “Anti-Mask” Twitter, I was entirely within what they called “Anti-Mask” while we self-identified as “Team Reality”… on a side note, is it ethical for researchers to study Twitter and call random subnetworks they don’t like “ugly buttfaces” or other derogatory titles which they don’t fit their self-description?).
Yet, I provided a specific platform to managers aimed to maximize adoption of masks for the state of New York in the current US political climate, a platform that sounded very similar to that used by Governor Cuomo shortly after our call and which may have played a role in assisting the very high rate of mask wearing in New York. I’m very proud of my effort, my relative resilience, and my strategic emailing & phone-calling to bob & weave through the warzone and get the model to the managers & help the people of New York to the best of my ability. It was not east to deliver that message, but I’m proud I did it.
Summer 2020: Sweden stole the show
Emerging from battle, having seen my predictions come true, and equipped with the hypothesis that later unmitigated outbreaks are unlikely to be as bad (on average) as the NYC outbreak of March-April 2020, I began to test my hypothesis. I plugged into the global COVID data streams and developed dashboards to test my hypothesis against conventional models that drove public perception and policy. After the spring of 2020, I consulted a variety of managers, from Broadway execs wondering about how they might adapt to COVID (quipping that COVID was a cruel Samuel Godot parody, “Waiting for COVID”), to managers in low-income countries like Rwanda. Low-income countries had cost constraints and were neither able to lockdown nor implement expensive test & trace policies which, again, one theory said was a valuable policy and my theory suggested costs a lot and only marginally slows the pandemic. During this time, I saw again the major institutional modelers from the UK and Harvard had provided materials to managers which estimated pandemic burden in these countries. Their models recommended lockdowns without adjusting for different demographics or accounting for very different economic and public-health risks facing these countries. Justin Silverman, Nina Schwalbe, Jeanine Condo and I discussed ways the pre-existing maternal-child surveillance systems of Rwanda could be adapted to lower-cost syndromic surveillance networks, I pointed to the feasibility of pooled PCR testing to lower costs where COVID confirmation was needed to provide costl treatments, and I was greatly pleased to see a team comprised entirely of African scientists published a cost-saving pooled-PCR testing piece in Nature.
In between calls, I made dashboards for testing the competing theories of COVID from the Spring of 2020. The dashboards I made are cool advances in epidemiological data-visualization based on a simple and intuitive concept. Imagine, instead of living in different counties experiencing COVID-19 epidemics, we’re all sitting in different carts on top of a hill. The hill looms below us and a cloud blinds us from seeing the bottom of the hill. We’re scared - how hard can we crash if we go down this hill? Imagine the only data we had was the altitude and velocity of all the other carts - could we learn about how far down the hill is and how perilous it would be to proceed without brakes?
As thousands of carts go careening down the hill all around the world, we’d see their velocities rise and their altitude fall. If they slammed on the brakes, the cart would stop at a fixed altitude and its velocity drop to zero - random, strong application of brakes would lead to carts stopped at a whole range of altitudes. If they released the brakes, we watch their velocity rise again and their altitude fall. Plotting velocity versus altitude-lost from the peak of the hill, we’d eventually be able to see a pile-up of carts at the bottom of the hill, a cluster of points that all decreased and saw velocities hit zero at a similar altitude. That’s the simple concept - a graph we’d use to see the difference between carts braking at random spots along the hill and carts piling up at the bottom of a hill. Instead of velocity, we use case growth rates, and instead of altitude-loss (a measure the loss of potential energy) we use deaths per-capita due to COVID. Deaths per-capita due to COVID were the most comparable daily-updated measure of cumulative incidence we had prior to the arrival of vaccines.
Below, I show these dashboards and two ways to view epidemics. I simulated a demo “NYC” outbreak in red to demonstrate this plot. Everyone and their mother in COVID saw cases over time as shown in the first column. However, plotting cases over time makes it difficult to align and compare outbreaks when they occur different times (e.g. NYC in March 2020 versus Miami-Dade in October 2020). On the right-hand side, the red line shows the trajectory of velocity (case growth rate) versus altitude-lost (deaths per-capita due to COVID) for the simulated NYC-like outbreak. Case growth rates decline continuously - again, that was what Levitt noticed - and deaths per-capita increase over time. The empirical NYC outbreak shows this same declining growth rate over “time” as deaths accumulate.
The red outbreak shows a simulated epidemic reaching herd immunity (strictly defined as the cumulative incidence at which cases peak. Not a “strategy” nor does herd immunity at some point in space or time imply immunity will be the same at other points in space or later times as immunity wanes). The purple, green, and yellow outbreaks all differ materially from the simulated NYC outbreak. (B) The purple outbreak shows a successful lockdown applying the brakes and ending the outbreak at fewer deaths per-capita. The cart stops before the bottom of the hill. (C) The green outbreak shows what would happen if the NYC outbreak were interrupted early, the brakes applied & then released, where we see a resurgence following the relaxation of non-pharmaceutical interventions. Towards the end of the second wave, the second wave would trace the “resistance line” in our dashboard defined empirically by the earlier, unmitigated outbreak. If the early outbreak was, in fact, truncated and stopped many people from dying, and relaxation by the Cuomo team would lead to resurgence & possibly 30K more ventilators needed, then later unmitigated outbreaks in other regions would look like the yellow line in (D) where, following relaxation of interventions or with the onset of seasonal forcing, the second waves would exceed the NYC line. Outbreaks breaking through this line would, at least for me, reject the hypothesis that the New York City epidemic resistance line defines an upper-bound on what to expect from unmitigated outbreaks.
These dashboards updated every day and gave me a cool, new view of thousands of outbreaks around the world. While many others were pulling down tabs to view a few counries at a time in Our World In Data, this dashboard helped me align thousands of asynchronous outbreaks visually and watch these thousands of carts all at once and instantly compare them to the NYC outbreak. I’m proud of these dashboards - I learned so much from watching them throughout the pandemic.
The figure below is an important snapshot of what happened in the Summer of 2020. The NYC line is in red, the black line is NY State, and the yellow line is Sweden. Sweden was the Twitter world’s pariah for not locking down, and conventional models estimated nearly 0.4% of Sweden’s population would likely die for cases to peak under unsuccessful mitigation efforts. At the same time, our laboratory of democracy was releasing & reapplying breaks as carts of state & counties went down the hill. To see how policy changes impact outbreak trajectories, the curves for each state are colored by the interventions in place at the time. We can watch the carts go down the hill, and color the arcs of their jounrey by how hard they were slamming on the brakes. Immediately, it’s clear that relaxation of shelter-in-place orders in US states coincied with a rise in case growth rates. For some states, reopening meant resurgence.
Sweden as a topic of great debate in the Summer of 2020. At the time, many countries were goaded by a very vigilant community of online epidemiologists into believing up to 0.4-0.6% of the population would die if they didn’t contain the virus. Sweden, however, wasn’t sure about the severity of the pandemic but was sure that such aggressive containment policies had a high likelihood of being worse than the disease, so Sweden chose to educate people on transmission and, albeit haphazardly, focus their effort on protecting the most vulnerable populations with the highest risk of severe COVID-19 outcomes. Sweden saw a sustained case growth rate leading to a peakafter 0.05% of its population, or 1 per 2,000 capita, had died due to COVID.
The low end of the “millions of deaths” scenario or 0.4% of people dying, is the far right side of each of these graphs. Sweden came nowhere close to the NYC line. US states like Florida and Georgia reopened, saw case growth rates rise, and then they peaked - their case growth rates hit zero - close to the same population fatality rate at which Sweden peaked. It was within the realm of possibility, then, that Sweden and New York State could define herd immunity thresholds for US state or Sweden-like countries. Such a hypothesis would imply that cases are unlikely to grow (at least this soon after a major outbreak) if the region’s population fatality rate exceeded the NY State population fatality rate.
Louisiana was an interesting case study. Louisiana had an early outbreak in March 2020 which led to a population fatality rate similar to that of New York State. In the summer, Louisiana relaxed its interventions and saw a later resurgence that rejected the hypothesis that NY state was an upper-bound for outbreak burden in US states. However, when analyzing the counties hardest-hit in Arizona, New York, and Louisiana with these same dashboards, I found what I felt to be strong evidence corroborating the NYC outbreak as a probable upper-bound (all these counties peaked, all the carts stopped, near the NYC stopping point). Incorporating this information, my updated model/hypothesis then implied to me - by August 2020 - that the US outbreak in the fall of 2020 across states was likely to peak somewhere between 300,000-400,000 deaths, not 2-3 million deaths.
Having experienced the very un-fun hostilities from the online mob and knowing these forecasts differed markedly from the narrative, I only shared these forecasts privately with a now fairly connected network of managers. I invited a few close & trusted watch this dashboard & thousands of carts roll down the hill as the fall 2020 outbreaks unfolded.
Serosurveys
There is one important biological detail that needs discussion, though. Following the NYC outbreak, a serosurvey found only 22% of New Yorkers had antibodies for SARS-CoV-2. For a virus with R0=2.4 (and probably higher given that R0 is based on what we now know was an underestimate of case growth rate), in a homogeneous population in which very person has the same number of people they contact & can infect every day, 60% of people would need to be infected in order for the population to reach herd immunity, and 22% is much less than 60%. How could NYC have reached herd immunity if only 22% of people sampled in grocery stores have antibodies?
For NYC to have reached herd immunity yet only 22% of people in grocery stores be seropositive, some combination of things must be true. (1) There must be some heterogeneity in transmission & susceptibility that reduces herd immunity thresholds (something Gabriela Gomes discussed elegantly and with similar frustrations about the online hostilities and disregard for inconvenient math) and (2) there must be some infections that don’t seroconvert.
Now, we know that both of these necessities for my hypothesis are true. There’s heterogeneity in transmission and susceptibility depending on age, race, place of work and more. There are also many patients who don’t seroconvert, as demonstrated by a Swedish T-cell survey, the Oxford-Immunotec survey of health care workers, and the later immunological mechanistic demonstration that pre-existing SARS-CoV-2 T-cell receptors recognizing RdRp can neutralize the virus and cause a patient to never test positive nor seroconvert!
In other words, there were some biological mechanisms required for the “NYC reached herd immunity in March 2020” hypothesis to stand, and the biological mechanisms required for the hypothesis ended up being true.
Zooming out a bit, the prediction of heterogeneity in transmission & susceptibility and T-cells being important players in immunity are cool examples of how this model-based theory, based on a sequence of conclusions from early case growth rates, infection fatality rates, venilators & ILI visist, pointed to biological hypotheses about SARS-CoV-2 epidemiology and pathophysiology. These biological hypotheses became facts & findings months after the hypotheses were first generated. Having this theory in hand gave me a way to predict the future, not just of velocities & altitudes, but also unexpected details about T-cells or vaccine-evasion.
I shared the figure above with a few trusted bipartisan connections who put it into the hands of managers. Shortly afterwards, Rand Paul asked Anthony Fauci about whether places in the NE might have reached herd immunity, to which Fauci replied that if Dr. Paul thought 22% was herd immunity, he was alone in thinking that.
The Narrative had spoken. I put my head down & got back to work.
Fall 2020: From the Declaration of Great Barrington to the Peaks of South Dakota
The Narrative’s predictions about June/July 2020 peaks were wrong because their growth rates were wrong, and their predictions of ventilator demand in New York were also off a factor of ~4x. Their Summer 2020 predictions of a dire 0.4-0.6% of people in Sweden dying for cases to peak were also - you guessed it - off by a factor of 8x. On the other hand, my theory predicted the March 2020 surge and set me on the path to estimating both the ventilator demand of NYC and the Fall 2020 burden much more accurately. In an ideal scientific community, one might think the conventional teams’ track record of screwing up the most important early calls of the pandemic would inspire more humility or perhaps motivate connections, collaborations, and discussions to integrate our models, connect our minds, and help us better understand what’s really happening. However, science, at least highly competitive institutions like Harvard and the Imperial College London, does not exactly select for humility, and I was a mere peasant in a Feudal system who had no luck getting in touch with those teams.
In early October, 2020, the Great Barrington Declaration was published. The GBD slapped the face dominant COVID narrative by advocating for focused protection. They argued that keeping our foot on the brakes at all costs, containing SARS-CoV-2 while we wait for vaccines, is not the only option and may be more harmful and unethical by causing public health harm in the service of public health policy. Don’t let anyone tell you otherwise: the GBD was a sane proposal that many scientists agreed with. In a world of COVID-myopia screaming “BRAKES!!!”, the GBD balanced the risks of COVID with the competing risks of our policies causing harm.As evidenced by Japan, Sweden, and Florida by the Summer 2020, the GBD noted that not only possible to focus protection on populations at a high risk of severe COVID-19, but that such a strategy was arguably more ethical. Given the known impact of lockdowns throwing millions of the world’s poorest people into hunger, focusing protection and minimizing the harm caused by NPIs could likely reduce global all-cause mortality & morbidity compared to slamming on the brakes even if it sends a hundred million poor people into acute hunger. Causing harm in the service of public health can harm the public’s trust of public health, and so, by the same ethics driving Hippocratic oaths, doing harm must be avoided. That was the GBD.
The Narrative did not like the GBD. The Great Barrington Declaration was met by a remarkable, merciless hostility. One would hope the scientific community would acknowledge some points, raise questions about others, and discuss the methods for protecting the elderly. Scientists doing their jobs could discuss and debate the proper mask-wearing and test-to-enter policies in nursing homes that, at the time accounted for ~50% of the deaths. We did not really have those discussions. Instead, the epiTwitter community went thermonuclear. This was expected given the mob’s prior treatment of Levitt, Ioannidis, myself, Gupta, Lourenco, Gomes, and others who deviated from the narrative. There was The Narrative and it was bad to change it. Only the priests were allowed to change the interpretations of the text, and any effort by people outside this inner circle to disagree or dissent or debate was met with a holy contempt. Conversations got so bad people were calling mitigation policies “eugenics” (I disagree). Folk said GBD proponents were “sacrificing grandma for the DOW” and this “let ‘er rip strategy” (a slogan written by people who hated the GBD) would kill 3 million people in the US. A chimeric culture of scientific beliefs, narrow political views, and online warfare was becoming less sociologically primordial and more of a clearly defined monstrosity.
Anthony Fauci and Francis Collins, it is now commonly known, wrote in an email that there needed to be a “devastating take-down” of the GBD. Many prominent Twitter epidemiologists from Harvard and Yale, who are well known to be connected to Anthony Fauci, advocated for containment. “BRAKES!” People like Marc Lipsitch advocated for containment polices, but had also consulted Pfizer as the company stood to earn tens to hundreds of billions of dollars from a vaccination campaign should the entire world slam on the brakes to contain the virus until vaccines arrived. This conflict of interest was undisclosed in a Washington Post article calling the GBD a “herd immunity strategy” and even on this Lancet article arrogantly claiming a “scientific consensus” , and on the John Snow Memo, a memo written in opposition to the GBD. Dr. Lipsitch reported support from Pfizer, Merck and other companies as far back as 2019, in late April 2020, in October 2020 , and again in December 2020. In other words, Dr. Lipsitch had an undisclosed conflict of interest when - coincidentally - orchestrating the devastating take-down desired by the heads of NIH and NIAID. Won’t someone please rid Collins of this troublesome fringe? Marc now co-runs the center for outbreak forecasting & analytics at the CDC.
During an ongoing global crisis, science devolved into a battle of memos & declarations with victory won by brutal populist propaganda tactics on mainstream media connections & Twitter popularity. The heads of federal agencies, who oversee billions of dollars in federal funding of a hierarchical scientific system full of scientists with competing ideas, had taken clear sides in scientific debates and their requests for devastating take-downs were followed by mass-media blitzkriegs. This, to me, was the surest example of manufactured consent during COVID-19. A narrow range of models led to a narrow range of policies in a narrow group of people, and these people projected The Narrative through channels of communication, from academic journals and mainstream media outlets to TV and Twitter.
Imagine if Pfizer had a drug that came it out in a time of peace, and someone getting paid by Pfizer went to the Washington Post and the New York Times to say the disease caused by the drug is more likely to kill you than it actually is. Imagine if scientists spoke up and said “wait a minute, I don’t think that’s necessarily true” and the head of NIH called for a “devastating take-down” of these dissenting scientists, and THEN the guy getting paid by Pfizer and a bunch of people closely connected with the heads of NIH and NIAID tell everyone they’re gonna die unless we all agree to the policies they propose, policies that happen to give billions of dollars to Pfizer.
That, to me, would be a very consequential conflict of interest, a swamp-like web of political connections that abuse the powers and privileges we grant to the head of NIH, NIAID, or even the CDC. Furthermore, based on adds from Pfizer that appear incessantly on my Twitter timeline (I keep reporting them as inappropriate), Twitter likely also receives money from Pfizer. Twitter became the forum through which lower-burden scenarios were declared misinformation and higher-burden scenarios enshrined as ‘consensus’. Coming from the guy who had credible evidence for low-burden scenarios, these pervasive conflicts of interest, in both our media industry and the scientists chosen to consult it, eroded my trust in the deliberative process by which scientists communicate the results and the world came to believe science.
In the Washington post article and Lancet article advocating for containment policies, all of which went viral on Twitter, the popular Twitter academics, including those with ties to vaccine companies, openly proclaimed they represented a “scientific consensus” when they estimated that millions in the US could die in an unmitigated outbreak. They claimed that we must do everything we can to contain the virus until vaccines arrive (“BRAKE!”). They advocated for containment polices AND sowed fear by continuing to use models that overestimated the risk of death, they rallied a false-consensus on models for which there were reasonable grounds to doubt, and they manufactured public perceptions to support the conventional teams’ policy preferences. Incidentally, all of these actions increased demand for COVID vaccines by increasing public fear of the virus.
Was the “consensus” right? Did The Narrative and the conventional models behind it have superior science? Or were their forecasts way off? The true referee of science is not the New York Times or Twitter, but the data. What did the data say, Fall of 2020?
States like Florida and South Dakota chose to do relatively little to prevent SARS-CoV-2 transmission. I drove all around the west to do some ethnographic surveying and double check my assumptions. I visited counties from Montana to New Mexico and corroborated my suspicions that many key archetypes out west - some big-ish cities with predominately conservative and libertarian populations - did not change their behavior much at all. These places would see relatively unmitigated outbreaks. These carts would have no brakes. The Narrative’s models estimated that a minimum of 0.4% of their populations would die just for cases to peak! In mid-August 2020, almost exactly when historical outbreaks of respiratory viruses would rise, COVID case growth rates rose, and outbreaks resumed. Velocity increased, altitude decreased, and carts went barelling down the hill.
What happened in counties that did little?
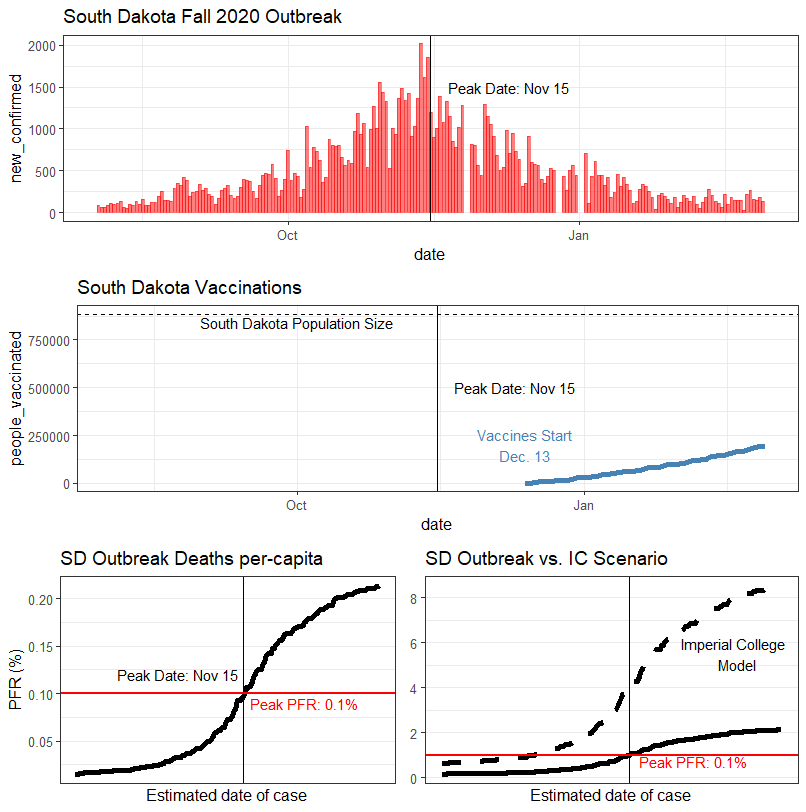
Fall 2020 was another important opportunity to test these competing hypotheses. I watched the world’s thousands of outbreaks play out in real-time using my dashboards. By the time the “devastating take-down” of the GBD began in force with the John Snow Memo, the Washington Post blitzkrieg, the Lancet “scientific consensus”, and a swarm of other cocksure content, places like Cook County were just about to see Levitt-esque decelerations in case growth rates that rejected the models underlying The Narrative’s COVID belief system.
Shown below are the fall 2020 outbreaks in the five largest US counties. Dots indicate where cases peaked, and dots are sized by the prominence of the peak. Notice on (B) how every single one of these outbreaks wiggles around the New York City line as it approaches its peak. These outbreak trajectories seemed to be squeezed & funneled to peaks near that of the NYC line. This observation provided early evidence that Fall 2020 outbreaks would peak near mortality burdens of NYC in March/April 2020. Carts are piling up, we might have found the bottom of the hill.
In panel (C) below, I switch the axes and show the deaths per-capita at the peaks as a function of time. In (C), the lower-bound for The Narrative’s “millions of deaths for cases to peak” scenario is shown as a dashed black line. Cases did not peak anywhere close to those scenarios. Across thousands of counties, cases peaked ~1/4 The Narrative’s lower bound. Instead of 40,000 ventilators there were 11,000… instead of 0.4% of the population dying where cases peak, the empirical truth was about 0.1%.
When we look closer at all US counties, this becomes more striking. Below, plots (A) and (B) show deaths per-capita 11 days after cases peak. For comparison, I include horizontal lines for the NYC March/April peak (red line), the Swedish summer peak (yellow) and the “40,000 ventilators”, “2-3 million US deaths” scenarios. Across all counties, cases tended to peak in-between the Swedish summer peak and NYC fall peak (corroborating NYC as an upper bound). (B) When we separate counties by whether or not they had mask-wearing mandates (a very bad, imperfect, but better-than-nothing proxy for mitigation intensity and horribly confounded by general attitudes towards doing anything about SARS-CoV-2), the states that had no mask-wearing mandates saw cases peak in December 2020 - January 2021 right around the NYC line, providing some evidence that some policies & behaviors correlated with mask mandates saved some lives. Millions? not really, but perhaps a few thousand to tens-of-thousands.
No matter how you look at it, 2020 was a no good, very bad year for conventional epidemiology teams. While they succeeded in sowing fear to increase demand for vaccines and manufacturing consent in the public to support more restrictive policies, their underlying models were unable to estimate the timing or magnitude of outbreaks - arguably the two most important things one should estimate in a pandemic. The models were wrong in the Spring 2020, the Summmer of 2020, and they stayed wrong all the way through Fall and Winter.
From the ventilators of NYC to the peaks of South Dakota, the conventional paradigm overestimated how bad the COVID pandemic would be by a factor of 4x. It’s worth stepping back and imagining the scenarios they simulated. For every person who died in the full hospitals of South Dakota, they estimated 3 more would have died in the streets. People would be dying of COVID waiting in line at the ICU, our medical system could completely breakdown, so we had to do everything we can to contain the virus. But… that scenario never happened. It was a hypothesis made from a model, and that model performed poorly throughout 2020 until it was basically rejected, in my mind, when 3,000 US counties from South Dakota to Florida all converged to the NYC outbreak burden, even (and especially) places that did basically nothing. I don’t know of a single high-income country with reliable death reporting that corroborates their model.
My models, and the theoretical journey they led me on like a hound on a game trail, estimated the timing of the NYC outbreak with uncanny accuracy down to the March 27th peak. They also estimated the sub-NYC burden of both summer 2020 and fall 2020 outbreaks. They estimated the median PFR at peak for 3,000 US counties including those that did nothing. Finally, they also anticipated the cool biology like T-cells fighting off SARS-CoV-2 so patients never seroconvert.
I felt like I could never share these findings without incurring the wrath of The Narrative. So, feeling I can’t publish my scientific findings and beliefs without incurring professional hostilities, I quit my academic position. I kept studying COVID, I consulted various groups as a COVID forecaster, and I used some spare time to try to help Native American tribes in Montana (as my models forecasted tries & rural communities of the state to be the most at-risk of devastating outbreaks). While many scientists did not pay significant costs for bad models, anyone planning a business or trying to make a living on the stock market did, so, believing the pandemic wave was over, I stepped back from publishing papers to do more consulting and forecasting COVID to bet on the stock market.
Since I ranted about COI’s with Pfizer, I want to elaborate for those unfamiliar with my arrangement why I believe my work in financial forecasting are not a COI. For compliance purposes, consultants like me aren’t privy to the positions held by my clients, so I have no way of knowing what news or policies will benefit my clients. In other words, while someone consulting Pfizer knows the products Pfizer wishes to sell and which policies will benefit Pfizer financially, someone providing forecasts to hedge funds is merely making detailed forecasts that are integrated into strategies without my knowledge of who owns what. Often, hedge funds incorporate information from multiple subject matter experts, and consequently my forecasts are just one star in a starry sky of expert opinions and assessments. The primary COI arising from this line of work is that I become deeply invested in being objectively right - if I make forecasts that don’t perform well out-of-sample, I will be perceived as less-reliable and my work less-valuable on this market.
As I mentioned before, I worked with one hedge fund for years prior to COVID, analyzing alternative datasets and forecasting with the humility one only gets trying to predict the future of the New York Stock Exchange. The forecasting tools I use in finance are uncommon in epidemiology, yet they were valuable for forecasting COVID and are the methodological reasons why I arrived at different results. You can see the interdisciplinary fun and value-added in the punny “IPO” analysis I did after the initial phylogenetic observation (not initial public offering) of B.1.1.7 and the use of “resistance lines” in the paper we finally wrote in 2021 covering all of the analyses shown above.
As I’ve shared elsewhere, I didn’t publicize or publish the research above in 2020 because of the hostility I witnessed from most epidemiology-enraged academics on Twitter and the conventional epidemiologists, including those claiming to be the “scientific consensus” that predicted a vastly different pandemic than I did. However, once vaccines had been disseminated and I believed I was at lower risk of triggering a mob saying I’d be responsible for deaths, I wrote about my dashboards and the thousands of carts rolling down a hill in a paper postdicting the 2020 outbreaks. In that paper, I say the quiet part about herd immunity quietly - I refer to “natural endpoints” as euphemism for “herd immunity thresholds” or “bottom of the hill”.
To support my claim that these were predictions & not post-hoc analyses that US states are likely to “peak” (see no case-growth) above 0.1% population fatality rates, below is an email I sent to a team in the CDC on August 17th, 2020, predicting “no growth” above 1/1000 deaths per-capita, or almost exactly the population fatality rate at which South Dakota later peaked in mid-November 2020.
I can’t un-see the body of evidence I was seeing or un-believe the COVID theory I for good reason believed. So, before 2020 ended, as cases peaked at 0.1% population fatality rates across the US as I’d predicted by August 2020, I left my postdoc to focus on forecasting stocks, providing COVID forecasts for anyone who’d pay for access to my scientific notebook, and providing these same forecasts and other brain power pro-bono to try to help public health authorities and managers pro-bono with a focus on supporting Native American tribes & rural communities.
2021: Getting vaxed, counting variants, and going for broke
While the fall 2020 was a bad year for conventional modelers, and the >20 million people who faced acute hunger due to COVID “BRAKE"!” policies disrupting international trade and cavitating supply chains, 2021 started off as an amazing year for vaccine companies and anyone who received funding from them. By the end of 2021, almost 5 billion people world-wide had received at least one vaccine dose, and Pfizer’s net income jumped from $9.1 billion to $22 billion.
I was fortunate enough to be on the original paper estimating the heightened transmissibility of the original variant of concern, Alpha, producing that punny IPO analysis I mentioned earlier. There was a difference of opinion between myself & other authors (and considerable uncertainty all-around) about what Alpha would mean for global outbreaks. To conventional epidemiologists, Alpha was a “lockdown buster” capable of transmitting so well that even lockdowns can’t stop it, and this would push unmitigated outbreaks to a mortality burden beyond what they’ve seen as well as cause some mitigated outbreaks to accumulate substantial mortality. To me, on the other hand, most places had acquired substantial immunity, and Alpha’s growth in the UK did not mean it would break through the substantial immunity in places like South Dakota.
Because I now had clients who depended on my forecasts, I had to stay honest and so I kept doing science with a sincere effort to test (disprove!) my theory. My theory of herd immunity at ~0.1% population fatality rates had been tested by a resurgence of cases in NYC in the Fall 2020. If NYC had herd immunity, and immunity lasts, why did it see a resurgence of cases in the Fall 2020 (albeit with MUCH lower mortality)? For these two things to be true, there must have been either weirdly timed seasonal forcing in the Fall 2020 (I had no reason to believe that) or some degree of waning immunity. Under this reasoning, Alpha would cause one of two things: either (a) Alpha is a lockdown buster that wreaks havoc across the US and causes major outbreaks in places like South Dakota or (b) immunity to SARS-CoV-2 wanes (this would explain the NYC fall 2020 outbreak) and perhaps Alpha will only cause outbreaks in places that had very early 2020 outbreaks.
Sure enough, places with major Spring 2020 waves and small Fall 2020 waves saw the largest Alpha outbreaks, while places like South Dakota, Montana, Arizona and Florida saw significantly smaller Alpha waves. This body of evidence gave me significant confidence that immunity wanes. Some of my confidence in these findings comes from living in Montana and knowing that, besides tourists and freight traffic (major contributors to Google’s mobility data in our states), life was more or less back to normal, with bars, restaurants and gyms having remained open throughout most of the pandemic and most returning to business as usual in the Fall of 2020. My ethnographic survey of the west anchored my beliefs in the hypothesis that many Fall 2020 US outbreaks were, in fact, relatively unmitigated. Combined with the data from Alpha, my analyses suggested that pandemic burden was lower than early models predicted, and that immunity wanes. That means immunity from vaccines will also probably wane. That, it turns out, was actually censored as misinformation - you could literally get banned from Twitter for saying that, yet that’s that the data were saying to me.
Our personal experiences as scientists may predispose us to believing things about the personal experiences of others. It’s tempting for people in the NE corridor or the UK to contribute these outbreak patterns to “behavioral changes”, as they lived in a world of lockdowns and closures. Meanwhile, I was rock climbing and floating down rivers in Montana with good mental health and not a care in the world. With these vastly different personal experiences came vastly different scientific assumptions about the role of “behavioral changes” in COVID outbreak burden - for me, the data above are disproof of the Narrative’s models, to conventional teams they reconcile the difference between model & reality by assuming behavioral changes account for the difference. These lived epidemiologal experiences from my travels around the US in 2020 are difficult to publish, but they also underlie my increasingly strong confidence in my model & now its broader theory. One of my favorite examples of diverse lived experiences during COVID was when I shared the 0.1% PFR findings with a good friend and colleague in the UK. I called him from Vegas, where I was climbing and rolling dice (admittedly, Las Vegas consumer traffic was shockingly low and masks were mandated indoors), and he was saddened because while I was climbing and gambling, people in the UK did not have Christmas.
I suspect these massive differences in the management we experienced play a major role in gut assumptions about the efficacy of our interventions in the pandemic, but since most epidemiologists live in large cities with strict COVID policies, it’s extremely difficult to convey to them that large swaths of Americans did not really care about COVID, including entire counties analyzed in my Fall 2020 work above.
While analyzing the Alpha data, Delta arose in India. My first hypothesis was that Delta was immunoevasive. Most conventional teams, however, estimated Delta to be, like Alpha, more transmissible. Transmissibility and immune evasion, like growth rates and infection fatality rates early in the pandemic, depend on one-another given the tools we use to estimate them. All we really see is the growth rate of cases for Delta being higher than other strains; absent other data, those cases are growing faster because of some unknown mix of higher transmissibility and immune evasion.
The conventional crowd, then, estimated that vaccines would prevent infection and, consequently, vaccination rates would determine outbreak trajectories. They estimated places with high levels of vaccination like US states would have much shorter outbreaks than places with so few vaccinations like India and South Africa. What happened, however, is shown below: India traced an arc of case growth rates over time and the key early outbreaks from South Africa to US states, followed it, despite massive differences in vaccination rates (nearly 0% in India ,<2% in South Africa, >40% in US states and >60% in the UK). If vaccines were the primary protection against infection & transmission, the UK should’ve had <40% the transmission rate of India and South Africa, along with a significantly earlier peak. But that did not happen.
Across the US, I watched Delta outbreaks unfold in two main ways. First, we needed to know: how well are vaccines reducing hospitalizations? When we plot hospitalizations per 100K, there was a very clear and expected negative association between hospitalization rates and vaccination rates. Vaccines reduced hospitalizations.
However, were vaccines reducing transmission? To study this, we can plot case growth rate (our best estimate of transmission) as a function of three types of immunity: (1) case-fatality-rate adjusted cases per-capita (using pre-Delta CFRs to estimate differential case-ascertainment across US states and use those different case-ascertainment rates to adjust the cumulative cases-per-capita to a common estimate of cumulative prevalence of mostly non-hospitalized cases from Delta), (2) Delta deaths per-capita, and (3) vaccination rate.
What we see are clear negative associations between transmission rates and the two metrics of natural immunity and no negative association between transmission rates and vaccination rates. Rather, as the fall outbreaks begin, places that had low vaccination rates and high deaths per-capita due to Delta, and therefore high immunity from natural infection, did not see a resurgence while places with high vaccination rates did. This suggested, by as early as July 2021, that perhaps vaccines don’t protect one against infection and transmission.
Saying that vaccines didn’t work, however, was anathema to The Narrative. Alex Berenson was banned from Twitter for saying it, and we all saw that. I knew it was not “okay” for me to say these findings before my eyes suggesting that vaccines may not protect against infection or transmission.
Only a priest could say such a thing. The CDC, in August 2021 finally said just that: in Provincetown, there was 100% immune evasion to infection (vaccinations did not protect people at all from testing positive).
Delta was immunoevasive, and the whole world went booster-crazy. Then, OMICRON, another variant of concern, was discovered in South Africa. The discovery of Omicron was followed by considerable debate about the extent to which Omicron was even more transmissible or immune evasive. At this point, with everything I’d seen to date, from the timing of outbreaks to the peculiar similarities in magnitudes to the immune evasion of Delta and the confirmation of unusual biological mechanisms like cross-reactive T-cells fighting off SARS-CoV-2 without patients ever testing positive or seroconverting… I had one last opportunity to test this theory before I lost my mind, went homeless, and babbled incoherently about carts, cases, and COVID up and down Route 66.
There was one last, important thing I wanted to estimate: what is likely to happen long-term with COVID-19? Under my hypothesis, NYC reached herd immunity in March/April 2020. Then, the Fall 2020 wave saw most US counties reach herd immunity, especially those all around where I live that did nearly nothing to prevent SARS-CoV-2 transmission. Then, Delta rose to prominence for exact reasons I don’t know… possibly shifting tissue-tropism, possibly changing growth rates in cell culture, all to plant a more solid foothold in the niche of endemic SARS-CoV-2. However, Delta - under this hypothesis - was the first glimpse at our second endemic cycle of SARS-CoV-2 depleting susceptible pools and, consequently, the relative sizes of the Delta wave to the Fall 2020 wave might carry information about future trends.
After all, immunity is not binary, but continuous, and adapting after every infection. The immune system is complex and honestly I don’t fully understand it. Noone does. I did advanced immunology courses in college, worked in a snail immunology lab for a few years, and in general kept up with the field because it’s reallt ty cool. Yet, I don’t fully understand it. All I have for this is biological intuition abouhe data we’re not looking at, much like the false-negative cases we weren’t looking at in repatriated travelers or the subclinical cases we weren’t looking at when counting ILI patients.
The biological intuition is that maybe, with successive outbreak cycles, most folks’ immune system gets better and better at resisting the infection and/or fighting off the virus with less severe symptoms. This is the same intuition behind a booster: more exposure = more immunity = better protection.
If we saw two nearly unmitigated outbreak cycles with Fall 2020 + Delta, then perhaps this can shine light into approximately how much better the immune system gets after an infection, and that could give us an early prediction about Omicron. The Delta outbreak killed ~1/2 as many people in the US as the Fall 2020 wave, so I estimated Omicron might likewise kill fewer than Delta (maybe ~1/2).
Let’s quickly back up in time to when Omicron was announced. When Omicron was announced, the world went into panic. Borders were closed to South Africa, vaccine apartheid and global inequalities were laid bare as people starved in Africa while people in the US and Europe locked their doors to get their guns & vaccines and hide from Omicron.
Among disruptions to global financial markets from Omicron, life insurance stocks dropped precipitously. The instant Omicron hit, the last fuck I had to give compelled me to run the calculations above and estimate how many people would die. Because life insurance companies report the cost of COVID-19 claims and the number of COVID-19 claims, I could back-computed life insurance companies’ claims into an earnings per-share hit on the company, and then used that to estimate a counterfactual earnings per-share in a world with no COVID-19 mortality. With these, I could approximately estimate how many people the market thought would die because of COVID, how many I thought would die, and see if the difference was worth investing in.
The difference was very much worth investing in. In a bold move that makes every financial advisor cringe, I put every penny I own into a particular life insurance stock that had strong fundamentals, strong float investment performance, and solid revenue growth (since people were scared shitless by doomsday models of their impending death, a culture of fear I did not contribute to). I went so far as to trade this on margin. Since betting every penny I own on my theory as it applied to financial markets in Omicron, the stock I picked is up 20% and the S&P 500 is down -16%.
As you can see above, the Omicron outbreaks in South Africa were followed later by early outbreaks in the US following a similar arc, putting Omicron into a similar category as the Delta outbreak (we wrote a paper about that). The data is in Fall 2020 wave killed 600,000 people, so I estimated Delta may kill 300,000, and Omicron perhaps another 150,000. Back of the envelope, this would give us 1.05 million US deaths by the end of Omicron. Now, at the end of the Omicron outbreak, there are 1.02 million US deaths.
Peace in a Post-COVID World
I still hold the life insurance stocks. I earned more from my COVID trading than I would’ve earned in many years of being a professor. Maybe I just got lucky… who knows? What’s slightly different about my luck compared to other day-traders who happen to have made it big is that my trades were based on my scientific study of a natural phenomenon, a natural phenomenon that was the dominant global macroeconomic disruption for two years. My predictions for the markets came from a scientific theory in a field in which I do research and publish novel findings, and my trades occurred in a social system with well-known inefficiencies in the diffusion of novel findings and other information caused by a field’s hostility towards novel theories like my own. Contrary theories were labelled misinformation, so markets didn’t get the memo.
How could market actors price in a scientific theory they never heard, because the main person studying it feared mobs & unemployment if he spoke up about it? My overall theory/history of COVID helped me predict a March 2020 surge in NYC, the lower ventilator demand of NYC, the March 2020 surge of ILI arrivals across the US, the lower-burden peaks in Summer & Fall 2020, the lack of an Alpha surge in places like South Dakota, the “immune evasion” of Delta in Provincetown, the cross-reactive T-cells aborting SARS-CoV-2 infections, and even the pattern of diminishing burden from successive outbreak cycles from Fall 2020 to Delta to Omciron.
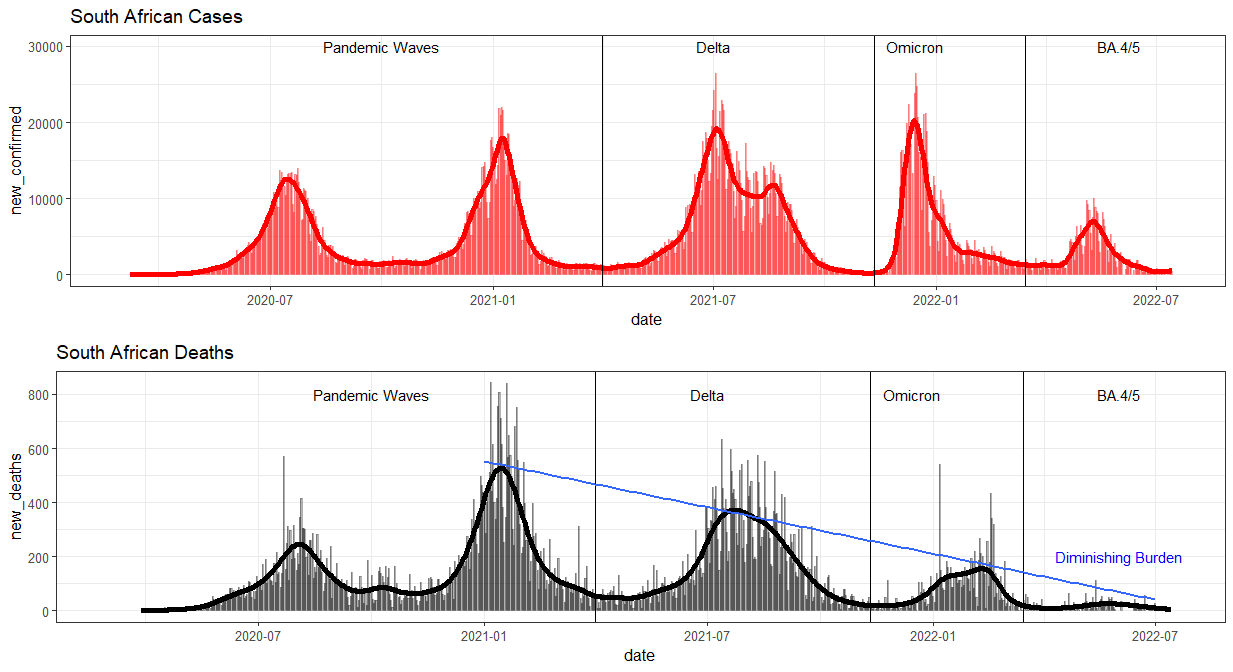
All of these predictions had macroeconomic consequences, from a Black Swan drop in March 2020 to the superior returns of life insurance companies since Omicron. Throughout the pandemic, I was eager to avoid being mobbed online for this unusually effective theory. After I quit academia, I depended financially on the accuracy of my predictions, so I continued to carefully and honestly evaluate the theory throughout the pandemic. I saw successive corroborations at major anomaly after major anomaly, each of which called into question the dominant paradigm driving the narrative of the COVID pandemic, and all of which combine to make me strongly believe my theory over the conventional paradigm that drove COVID narratives throughout this pandemic.
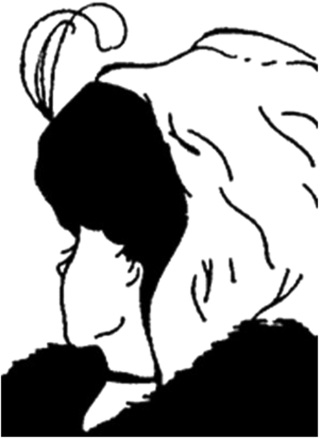
To this day, the Imperial College model is behind a counterfactual “millions-of-deaths” scenario estimate that 20 million lives were saved by vaccines. I don’t believe that number. I believe our best efforts to contain the COVID pandemic largely failed except in a few predominately insular countries. At the end of the day, not only did most places see the mortality burden of relatively unmitigated outbreaks (the real-world counterfactual counties among us), but our reactive, myopic caution caused harm.
Our reactive travel & trade restrictions, coupled with the fear sown into the hearts of civilians, may have helped the US and Europe to delay the inevitable 0.1% PFR for cases to peak in the pandemic wave, but it caused >20 million people to face acute hunger, threw >100 million kids into multidimensional poverty, and caused other “softer” forms of economic harm like surges in unemployment, defaults on debts & mortgages, and other real economic harms that hurt real people. Our school closures caused a highly inequitable rise in dropout rates and decline in educational attainment, catastrophic declines in math & reading scores, a rise in domestic violence, a rise in depression, and a persistent rise in all-cause young-adult deaths (mostly from overdoses) similar - in percentage terms - to the very worse rise seen in geriatric patients during the peak of the Fall 2020 wave.
My policy views on COVID stemmed from a mix of my models and the evidence I saw in trying to test them, and my own personal experience as a hard-of-hearing guy who grew up in the school-to-prison pipeline. If masks were mandatory when I was in school (as opposed to encouraged with flexibility & awareness of when they might not be ideal), my educational attainment would have suffered, so I opposed mask mandates (as well as vaccine mandates) and supported educating people about how masks work, recognizing there will be many important exceptions in classrooms and offices and stores all around our country. I felt we had to trust people to solve this problem with social norms instead of legal force. If my high school were closed, many of my friends in abusive homes would be beat and probably drop out of school, my friends whose parents smoked meth would not be able to focus (to say the least), and my friends who were struggling would not get the mentorship or the social support they needed to stay well. They would sit at home, drinking or doing drugs, and many might never be able to snap out of it to regain the tracks they were on for happier, healthier, more fulfilling lives.
I didn’t like lockdowns because I felt they were an unattainable ill-conceived idea. Even if we had lockdowns AND a massive social safety net to subsidize labor and pay everyone to sit at non-abusive homes watching TV and doing their math & reading homework, this wouldn’t solve the problem of people outside our borders. Most of the world is outside our welfare net, and I feel it’s cruel to recruit the world into our network of global travel and trade only to contract the network during an emergency, leaving the global poor to starve with no US citizenship welfare. I especially didn’t like lockdowns proposed for the west in places like Navajo Nation when coming from epidemiologists in the NE corridor who had never set foot on tribal lands or spent the night in a home on the rez. Growing up in New Mexico with close family & friend ties to many tribes in the state, I knew that lockdowns were unattainable for these communities - most people on-rez lived in large, multigenerational homes with essential workers where elders are the primary caretakers of kids, and many of these desert reservation homes lack running water. A lockdown would mean Harold goes to work every day and gets water at a gas station, gets sick, comes home and gets everyone in the home sick. What’s worse, what takes 7 steps of paperwork to reimburse someone off-rez takes 70-steps on-rez, and so how the heck would we subsidize housing to help people re-assort in a way that prevents transmission?
The concepts of the Great Barrington Declaration resonated deeply with me. Not only was the world being goaded into policy action by massive overestimates of how many would die, but they were also not being told the costs of COVID policies nor did they have the resources or support to feasibly contain COVID without causing massive, inequitable, and I believe unethical harm to the people starving outside our borders. However, the people I know love the people they’re with on a day-to-day basis, and it seemed feasible we could reduce all-cause harm by arming them with concepts about how transmission works, how masks work, which masks work, and some models for how one can protect the vulnerable.
I implemented the GBD “focused protection” concepts time and time again, and slogans like “protect the elders”, slogans ridiculed by epidemiologists from the UK and New England, resonated with tribes and became the motto in places like Navajo Nation as they adopted multi-pronged strategies that drew more heavily on their Tribal values and circumstances.
I’m sharing all of this because a key feature of the mobs during COVID has been an unbelievably rash jumping to conclusions about a person’s expertise and/or character. I was called “anti-mask” despite being one of the original people who encouraged masks in a critical, emergency context that led to mask adoption. I was called “responsible for deaths” by academics left & right because I had a model that more accurately predicted fewer deaths from COVID and encouraged reducing deaths from famine, supply chain disruptions, and other collateral human-health harms from COVID policies. I was called “far-right Trump-supporting Libertarian” by colleagues and told I was “not an epidemiologist”. Yet, I am quite adept at epidemiology and quite left of center, deeply & forever committed to reducing global & national inequalities in health, from the starvation of people in Africa to the public health policies of underrepresented and historically marginalized populations in America.
I know some people may jump onto Twitter and see some old tweet I blasted during the warzone of COVID-19, looking like the linguistic/social-media equivalent of Rambo unleashing a machine gun at ‘Nam. I apologize for where I was indelicate. I apologize for the many times I got angry, usually because of what I perceived as elitism (because I was just a postdoc at a state school), credentialism (that I was not an epidemiologist), alarmism (that millions in the US would die), tribalism (that anyone associated with the GBD is bad), and even the weaponization of research ethics to take-down scientists (e.g. as Gavin Yamey, Carl Bergstrom, and others had done trying to take down Ioannidis by saying his study of twitter epidemiologists violated an unwritten and non-existent research ethics “rule” they just invented about not publishing twitter handles in a paper). I apologize for coming off as strong - in my defense, me coming off strong felt like me spitting against the sea of hostility that came towards me when I tried to share my methods, analyses, and forecasts.
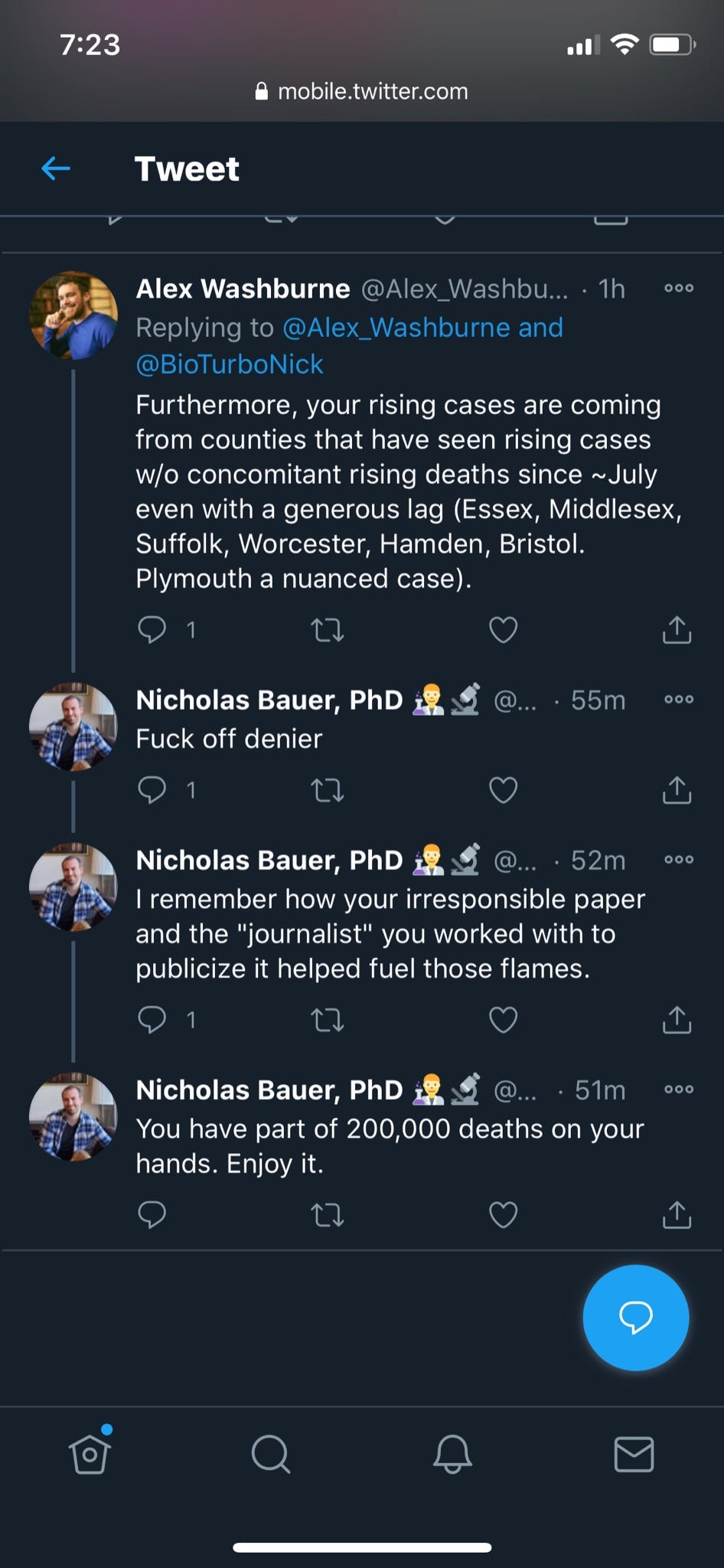
After almost two years of frustration and battle, I left my academic job and made a small fortune betting every penny I own and every dime I could borrow on my theory and its implications for the stock market. If I were less interested in human affairs, less stubbornly committed to making the world a better place, and less in love with science, I might just retire in Bozeman and go fishing every day. However, I’m turning my pain from COVID into purpose. I’ve co-founded a company building a social medium for science. I hope this medium can be a place where scientists can get away from the mobs of Twitter, rediscover the elegance of humility, and find a platform better designed to share scientific information in times of peace & times of pandemics, war, famines and more. I want a safe, fun, and inclusive environment for scientists to learn from one-another, to give each other the benefit of the doubt, and to function as a community even in times of crisis.
I’m excited - I love science, I care a lot about it, and I think we’ll all be better if we get off places like Twitter that turned men into monsters during a global emergency. We’re all on the same team, and I hope that this medium can provide places for smaller, intermediate results and cool figures like the ones I’ve shared here that were so influential in my own understanding of COVID-10.
That’s all. I hope this is the last thing I ever write about COVID. If I’m to be judged for my actions during this pandemic, let it only be by people who’ve read this far.
I love you all.
Edited to add:
After reading this article, my mom wrote me asking: “What lessons have you learned?”
Peer reviewed papers can be wrong. Unpublished pre-prints can be right. This is something I as a scientist have known since undergrad, where we discuss what historical scientists got all wrong. However, many in the pandemic exalted ‘peer review’ to a position of authority that it doesn’t deserve, and tried to undermine ‘pre-prints’ by relegating them despite their being correct and preceding peer-reviewed literature by 6 months, a year, or sometimes longer.
Sometimes the experts are wrong. This isn’t a knock on expertise as a concept, but rather this is a reminder that no matter how “expert” one may feel, humility is a necessary virtue in science. Today’s expert’s are tomorrow’s amateurs, and tomorrow’s experts are today’s outcasts.
All science is interdisciplinary. Disciplines are artificial lines delineating a contiguous space of higher thought. These disciplines are used to define departments, journals, and the allowable range of acceptable experts on a topic, but in this process of atomizing people into disciplinary cages, we Balkanize our knowledge economy and prevent the exchange of ideas across disciplinary borders. Disciplines can produce a territoriality towards epistemic trespassers, as I experienced when called “not an epidemiologist”, and in this way incumbent powers within disciplines try desperately to hold the reins of power by excluding others whose ideas could revolutionize their field.
We all need to be a lot kinder. Our society is getting revved up on social media and we are going for each other’s throats. With every merciless mob galvanized to define as forever immoral those who disagree, the set of people who fit into our shrinking moral space will dwindle until we are all alone in an intolerable, intolerant world. In addition to corroding the social fabric that holds us together, our demonization of each-other has a very real, chilling impact on folks’ willingness to say something should they see something in an emergency.
The honest scientist must never give up. I had many opportunities to give up, to retreat away from the disciplinary territoriality of prominent epidemiologists and stay in the lane they believed I belonged. However, I honestly care about the truth of COVID, the real timing of surges and the real outcomes of unmitigated outbreaks. While I retreated in frustration at times to put my money where my math is in the NYSE, retreat is an opportunity to plan a counterattack (I believe I got that concept from the I Ching). When you’re banging your head on a wall, look for a door. When you have an insight that you know others are not seeing - when you see the young lady blink or the old lady talk to shift paradigms on the optical illusion above - it is essential that you never give up, that you share your work assertively.
I shied away from sharing my work publicly, but I still injected these forecasts into managers’ inboxes through a variety of doors that I found - key allies who gave me the benefit of the doubt, key colleagues who were interested in my methods, and key friends connected with people in positions of power. In a world of doors, there is no such thing as a wall, only a stubbornness that blinds us to alternative paths - be like water, go around the rock, and find ways to maximize your effectiveness conditioned on your primary limitation of being just one person in a world of billions.We need a social medium for science. Our current platforms for the exchange of ideas and information in science are clumsy, inefficient, and they reinforce pre-existing power structures to slow the diffusion of discovery & innovation.
I’m devoting the next chapter of my life to improving this. Turn your pain into purpose, let your departure from the rut be the first day of your path. The socioscientific inefficiencies that pain me from COVID are the purpose that drives me to create a better platform for the next generation of scientists managing the world during a crisis. My departure from academia defines a new path in my life, a path less traveled by that I hope can make all the difference.
I hope on the contrary that this will not be the last article that you will write on the covid. It will take many more years to analyze what happened and recognize mistakes. It's very important for next time
Hi Alex, this is an incredible piece. I’m devouring “every word”. I’m halfway through and will pick up the rest tomorrow (1am in the UK).
Just wanted to say that this is a really valuable story that you are telling. So sorry for the hostility and silencing you’ve experienced. Thank you for all you’ve done and the person you’ve chosen to be through this time.
The more I read your work and journey, the more I love your heart, and mind. Keep being you.
(As you’ve so well articulated, when you see something, you have to say something (your precise wording is better). I’m speaking up in my own little corner in my way, part of which includes sharing narrative-questioning content, such as your latest CSI (“Totality”) post on Facebook.)
Best wishes,
Paul